The following is the skeleton of a keynote I gave at Pycon Poland
in August 2017.
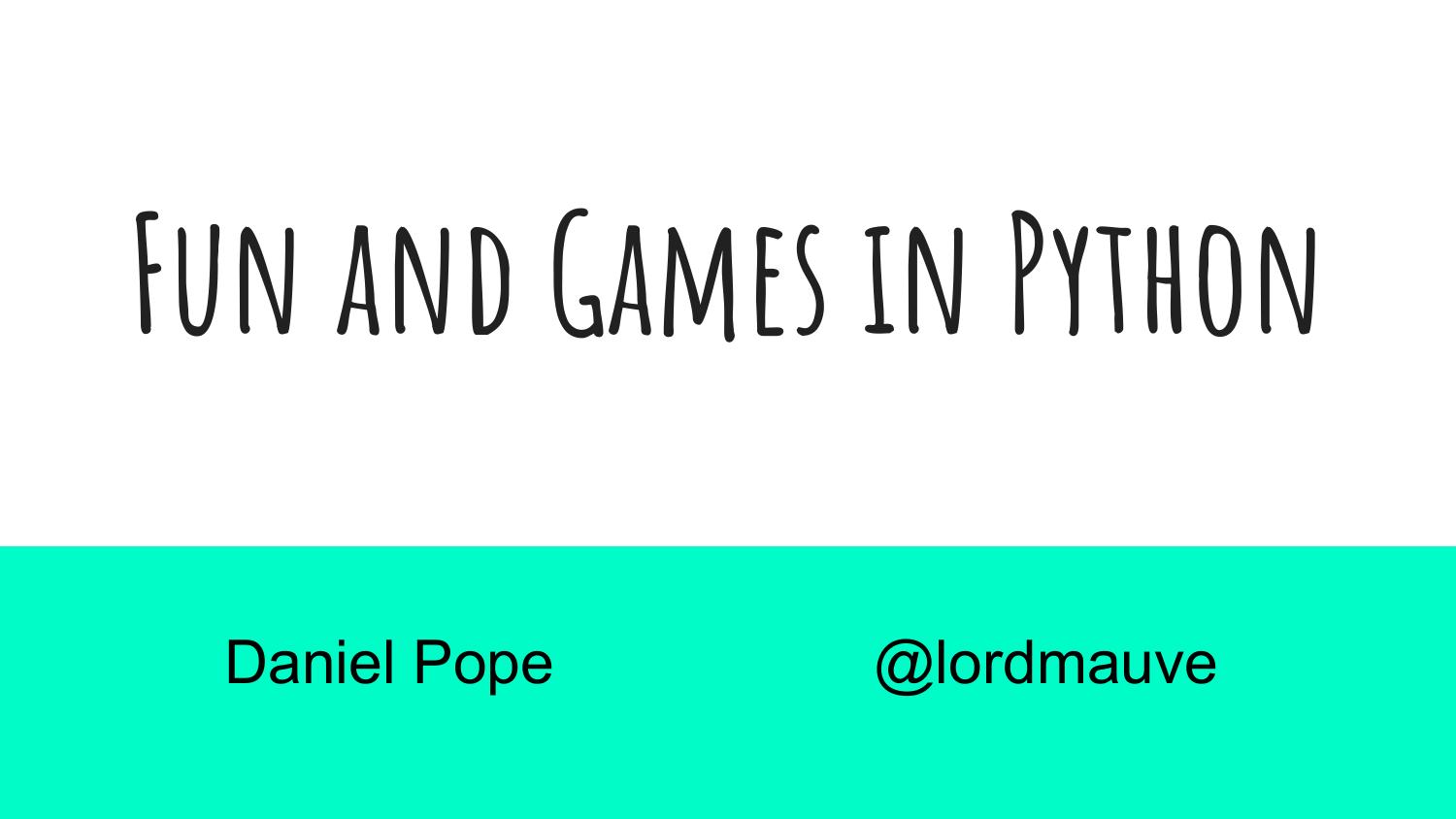
Fun is powerful.
Fun motivates us. I play video games, because it’s fun. I program Python
because it’s fun. I do not program PHP, because it’s not fun.
I think that probably Python exists, at some level, because Guido
thought it would be a fun thing to create.
I’m going to tell a story about fun - something of the history of
programming video games in Python.
Programming games in Python? Isn’t Python an objectively terrible
language for programming computer games? It’s so slow! Well, I think you
know that that isn’t the whole story. Python is used for crunching some
of the biggest sets of data in the world, in applications where clearly
performance matters.
How is it possible that such a slow language can be appropriate for big
data crunching… and games programming? To find the answers we have to
look back 20 years, to the age of Python 1.x.
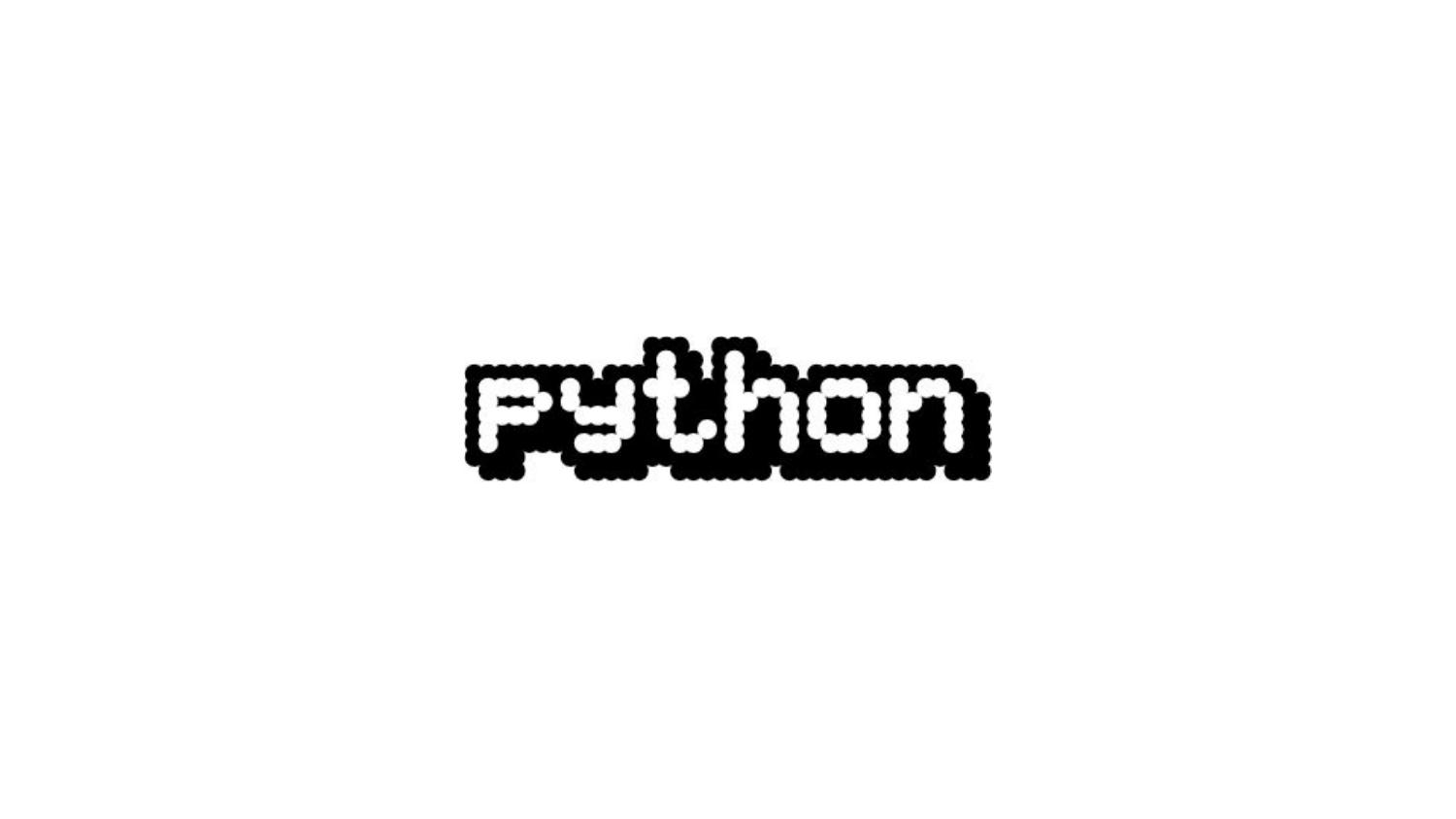
For big data crunching, that story began in 1995 with a library called
Numeric, written by Jim Hugunin, which was later rolled into something
called “numpy” by Travis Oliphant.
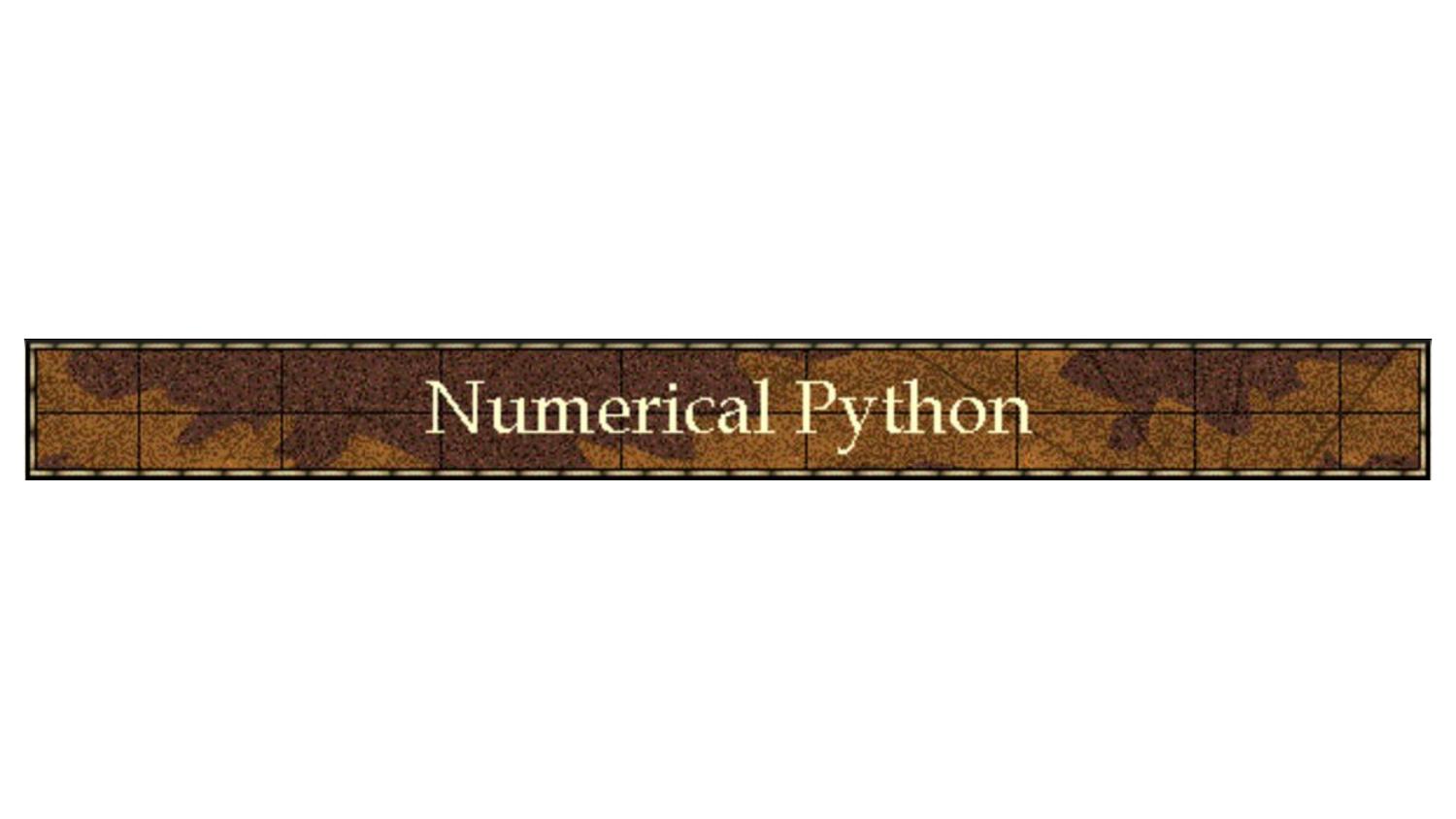
Jim Hugunin was also one of the people who was responsible for Python’s
first OpenGL library, PyOpenGL, with Thomas Schwaller and David Ascher,
which dates from around 1997.
OpenGL is an API for writing 3D graphics applications, including games.
However, OpenGL is not sufficient on its own for writing games; it lacks
support for things like sound, and creating windows and handling their
input.
In March 2000, Mark Baker started working on bindings for LibSDL - the
Simple DirectMedia Layer. SDL provides capabilities to render graphics,
but also support for creating windows, handling input devices, and
playing sound and music.
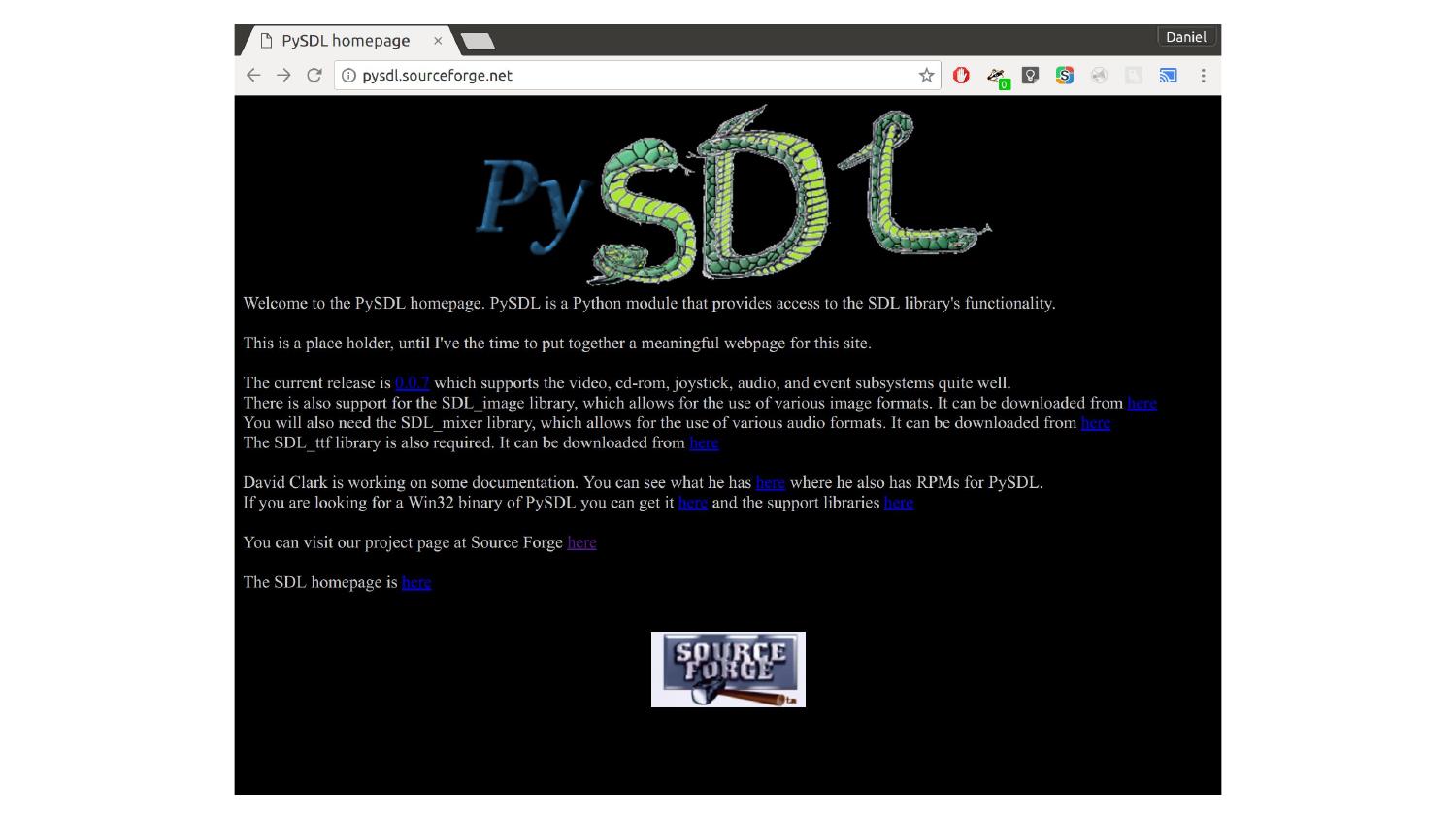
Later that year, Peter Shinners refactored this into a library called Pygame,
which was released in October 2000, the very same month that Python 2.0 was
released. It was now possible to write graphical games in Python!
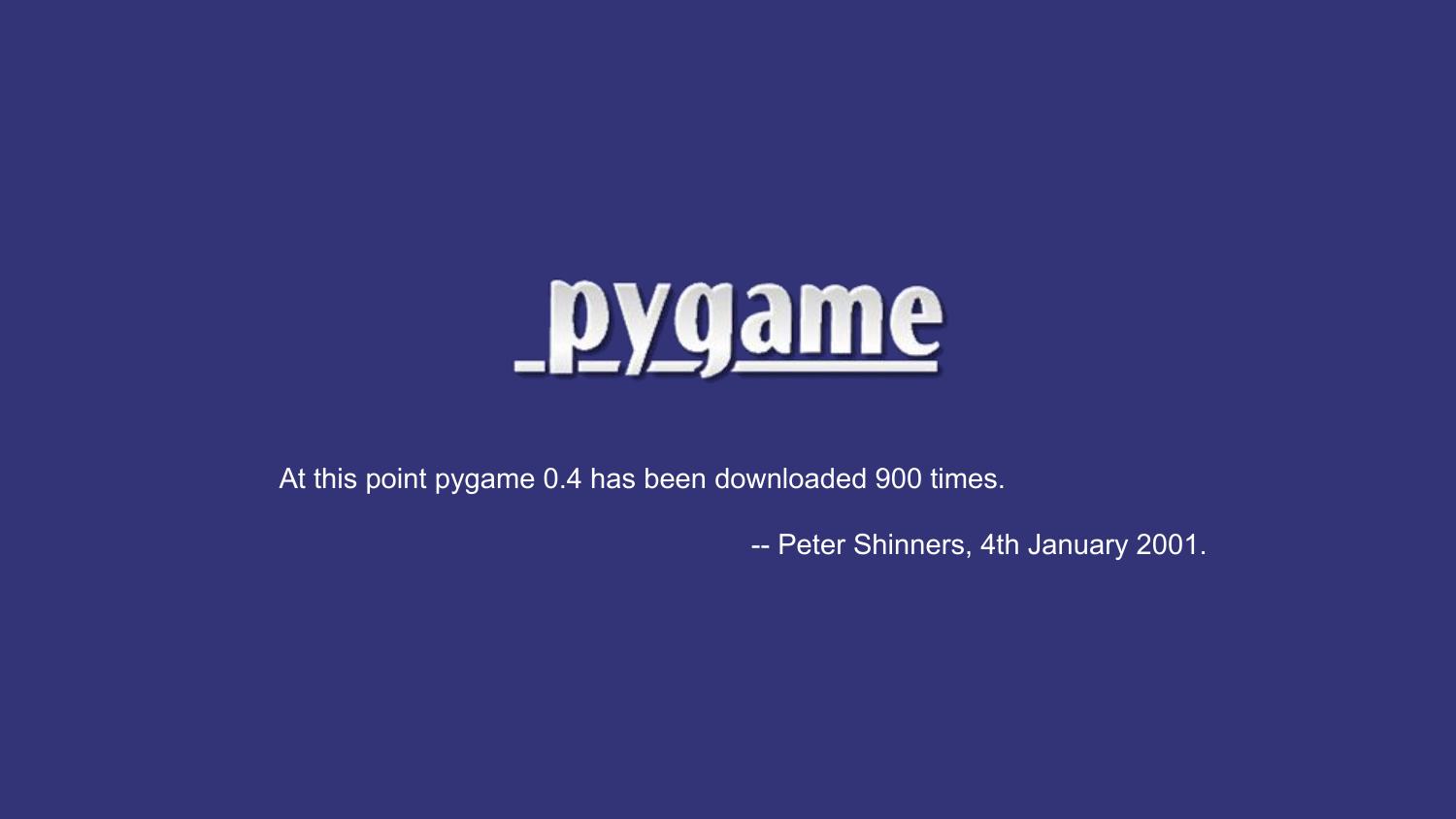
We’ll take a look at some Pygame code a bit later.
So this is the answer to how we can write fast, graphical games in
Python. We can do it because we use Python to drive fast, lower-level
libraries.
It was in about 2003 that I encountered Python for the first time. I had
already taught myself to program games. I learned Java at university.
I’d written a few Java games, and I experimented with writing games in
C++ with DirectX, OpenGL and SDL.
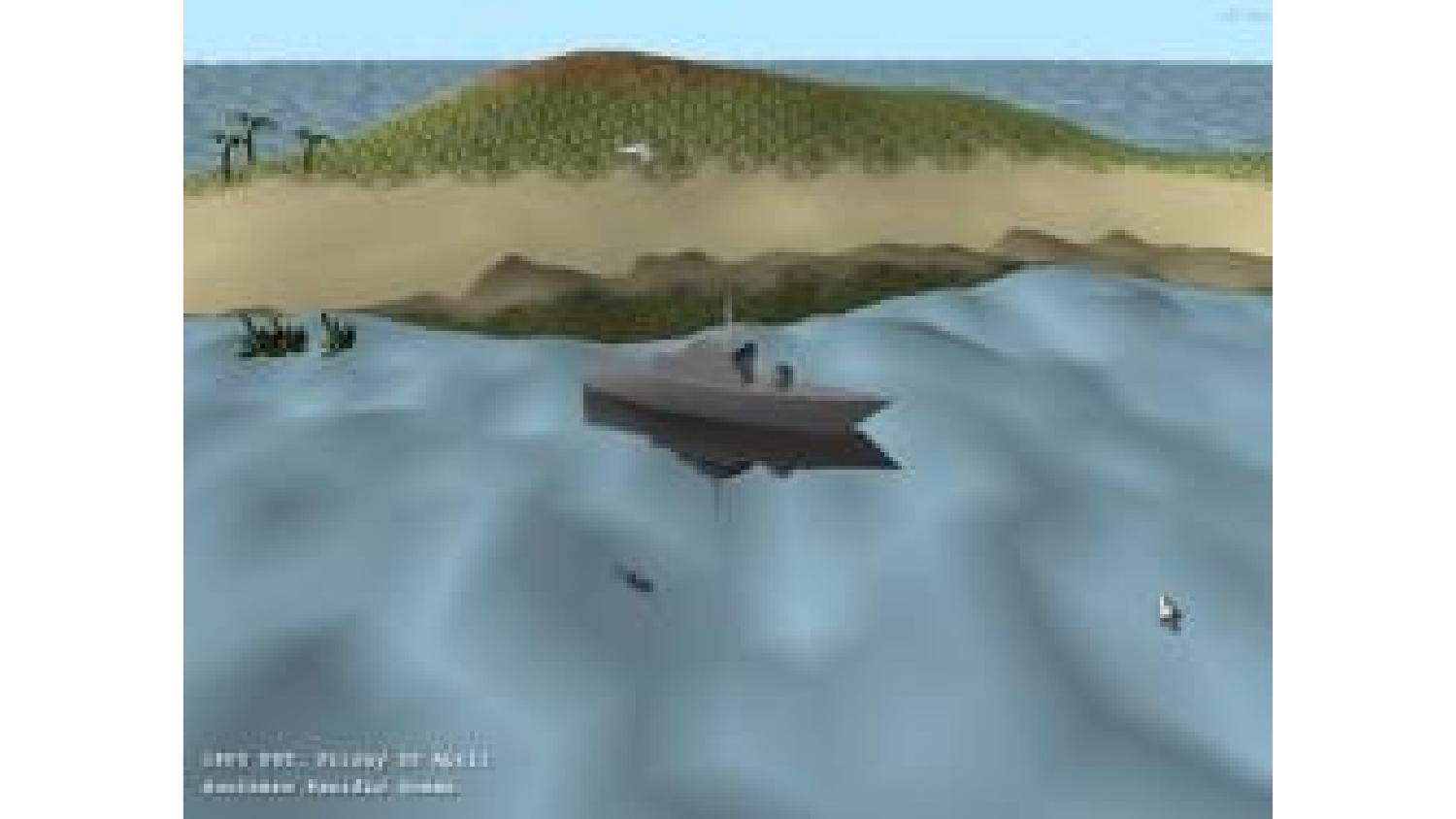
But programming in those languages was
slow and painful. Python was a fun language to program in! I was working
as a web developer/sysadmin person, so I was primarily using Python for
that at first. But of course, I eventually tried writing some games with
this “Pygame” thing. And I was amazed! I had written half of a
complicated puzzle game and drawn tons of graphics for it before I
realised I hadn’t really considered how to make it fun.
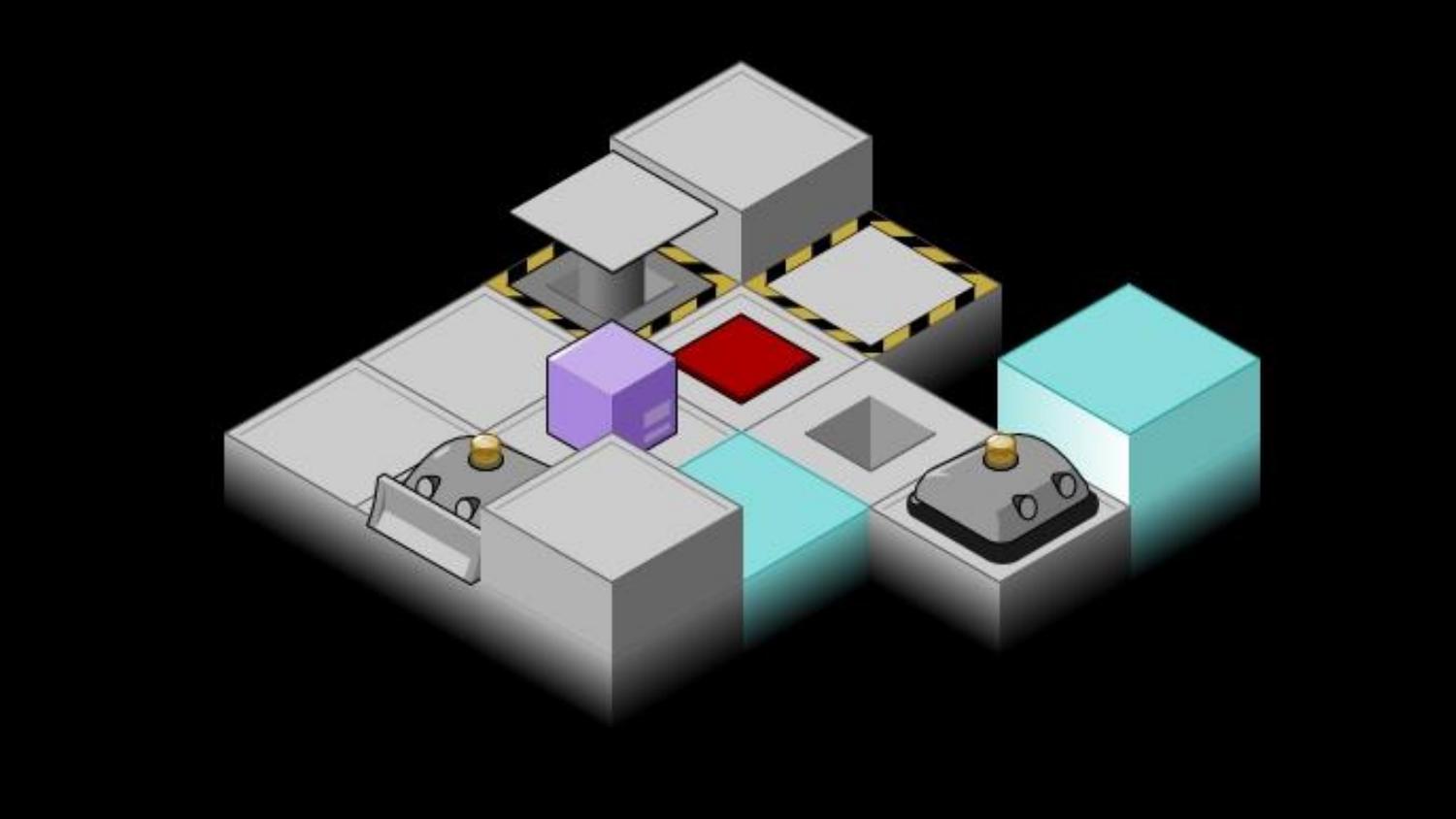
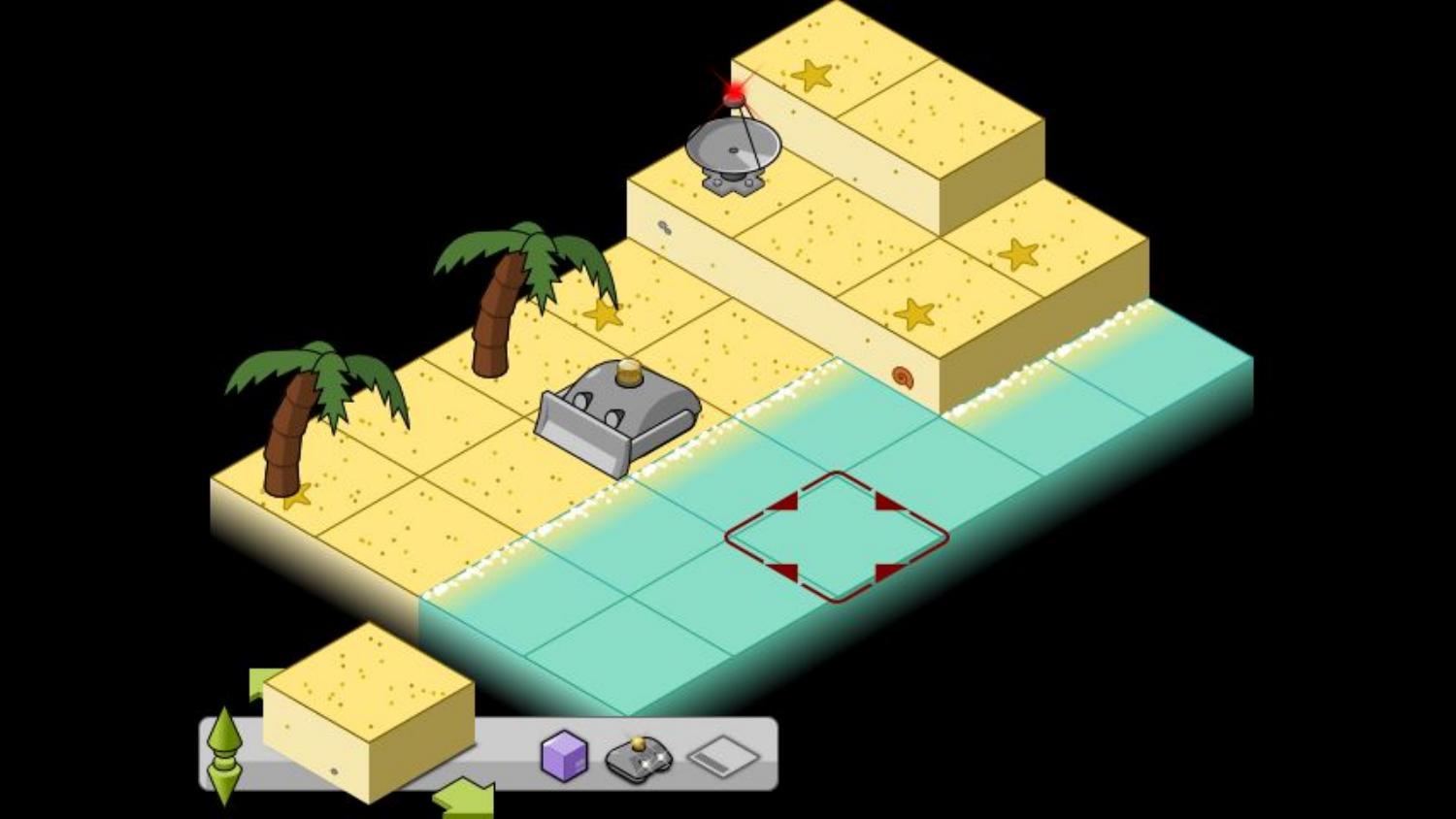
When showing my half-finished game off, somebody recommended I should
try my hand at a Python games programming contest called “Pyweek”.
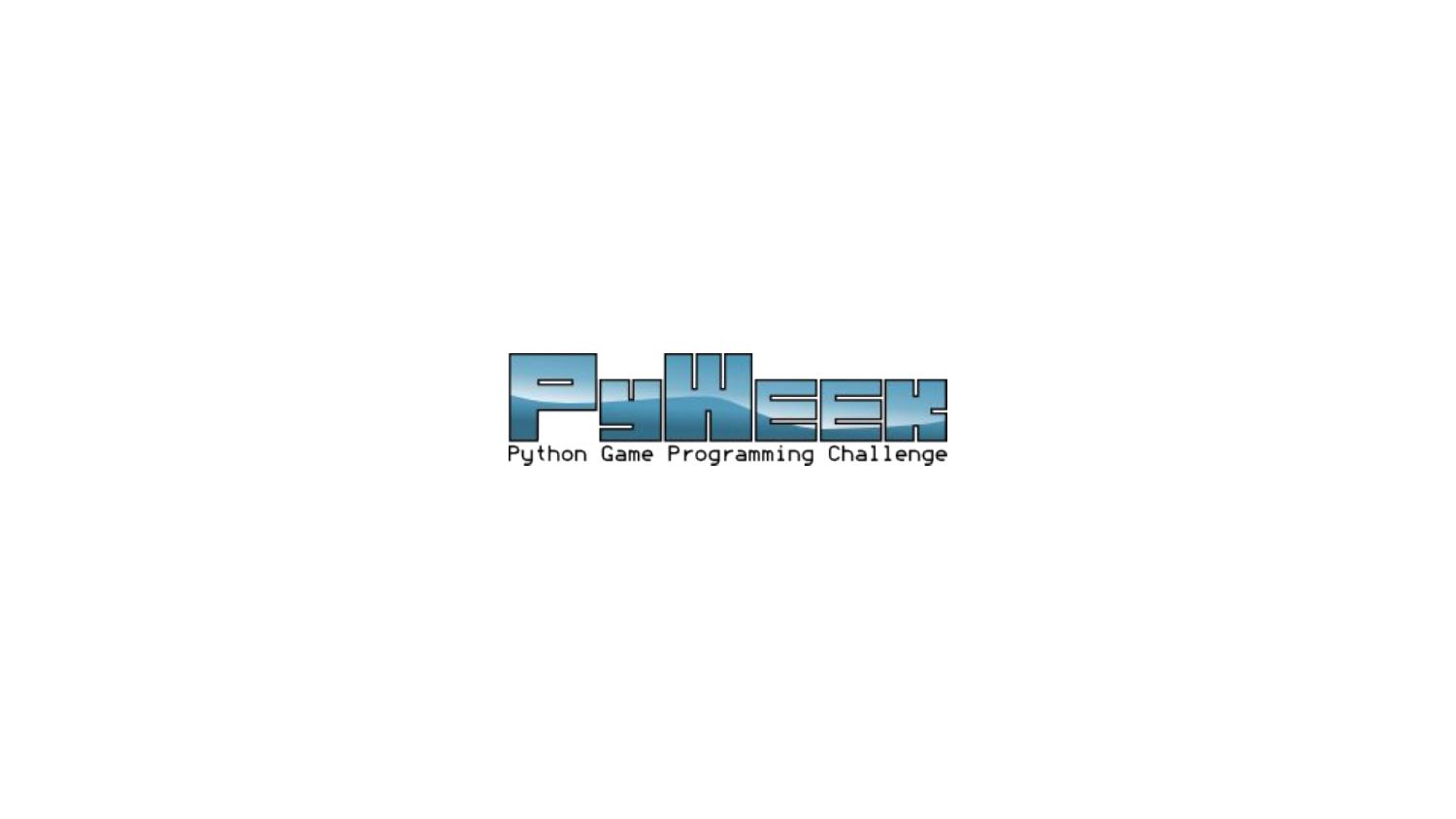
PyWeek was started by Richard Jones in 2005. Richard is perhaps better
known as the BDFL of PyPI - originally the “Cheese Shop”. He also wrote
the Roundup issue tracker that powers bugs.python.org.
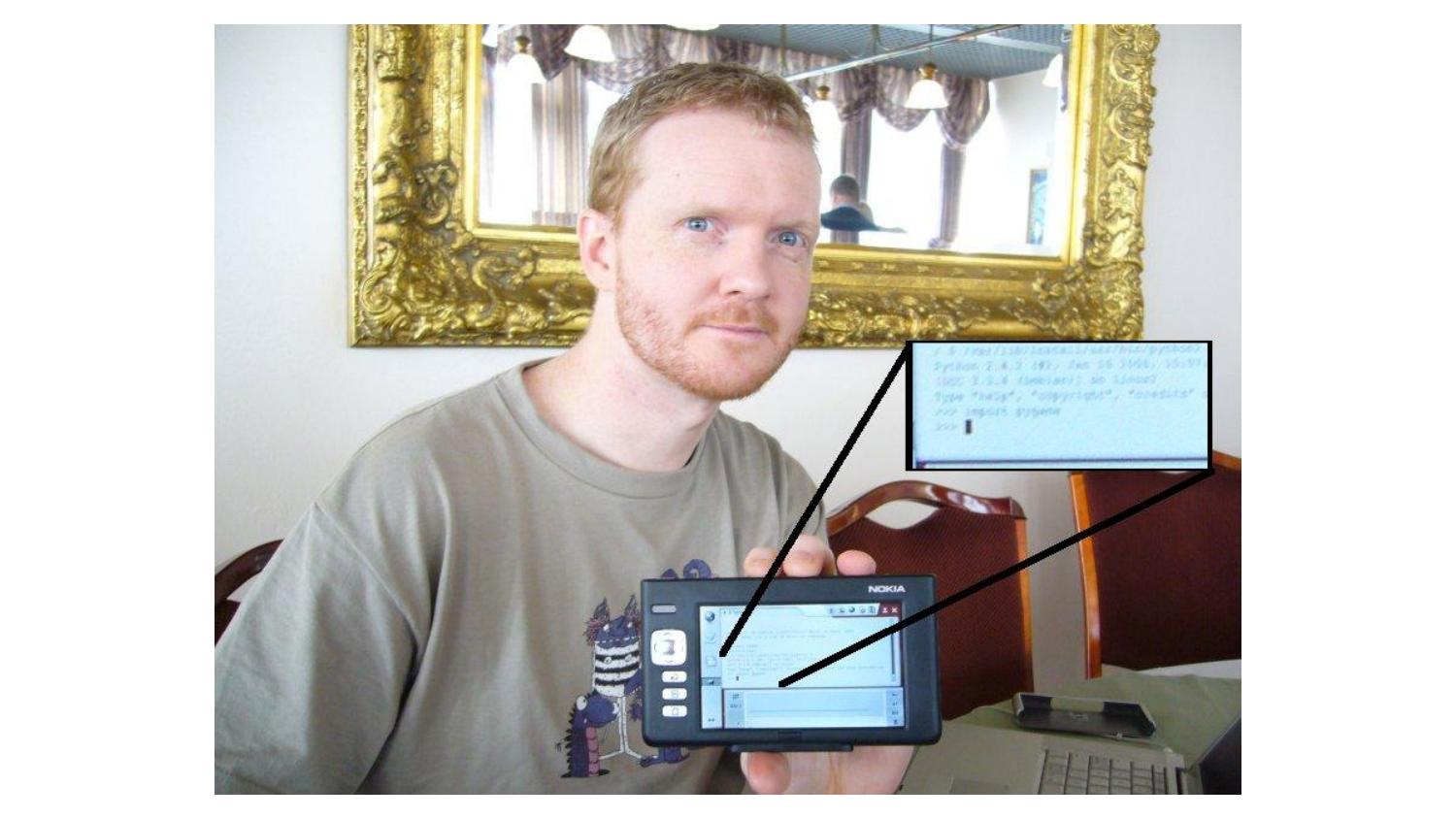
PyWeek challenges participants to write a game from scratch, in Python,
in exactly a week. Games have to be written from scratch - which means
that they may only make use of published, documented libraries created
at least 30 days before the contest starts. You can enter as an
individual or as a team; there will be a winner in each category.
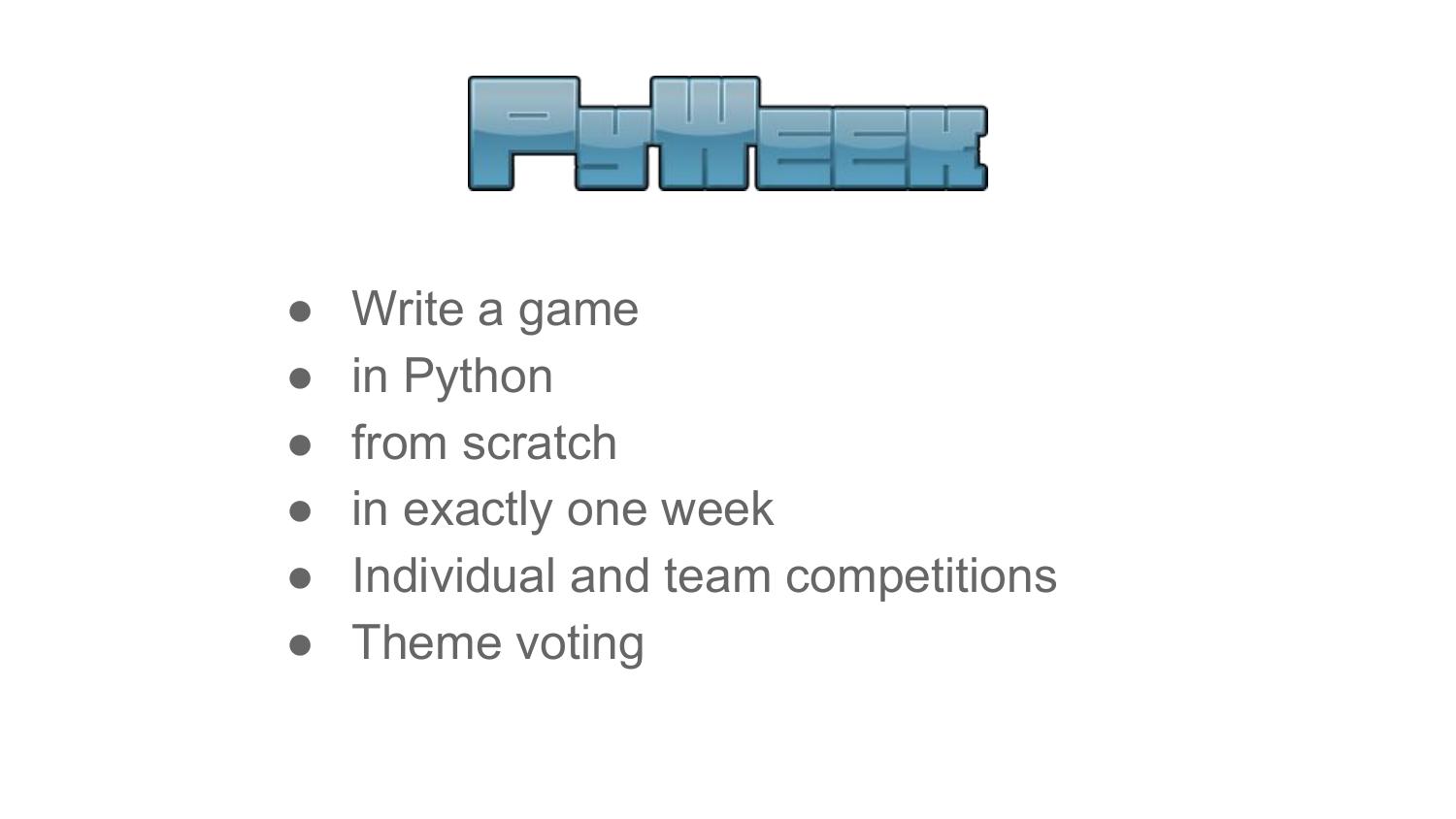
The week before the competition, five possible themes are put up for
consideration and votes are collected. At the moment the contest starts
- always midnight UTC - a theme is picked using a run-off system, and
coding begins!
During the contest, you’re able to write “diary entries” about your
progress using the PyWeek website, and you can also follow along with
the other amazing projects that are being created on the same theme.
This is also a forum to discuss your approaches and problems and get
feedback and tips.
After just 168 hours of coding, the contest is over! You upload your
game, and probably take a well-deserved day or two’s rest. Then everyone
who entered a game can spend two weeks playing and reviewing the
entries. Entries are scored on three criteria: fun, production and
innovation.
Fun is of course measured in how enjoyable the game is to play. The
innovation score measures whether the game includes original ideas, in
terms of mechanics, theme, plot, control system or whatever. The
production score is given for the overall quality of the presentation:
graphics, sound, and music, tutorials, title and game over screens… and
you might lose production points if your game contains bugs.
Of course that PyWeek was a success, and has run roughly twice a year
since then. The latest competition was the 23rd, and the 24th is
happening in October.
My first Pyweek was Pyweek 10 in 2010. In that competition, the theme was
“Wibbly-Wobbly”. I created a ninja fighting game set in a wibbly-wobbly bamboo
forest, like that scene in Crouching Tiger Hidden Dragon.
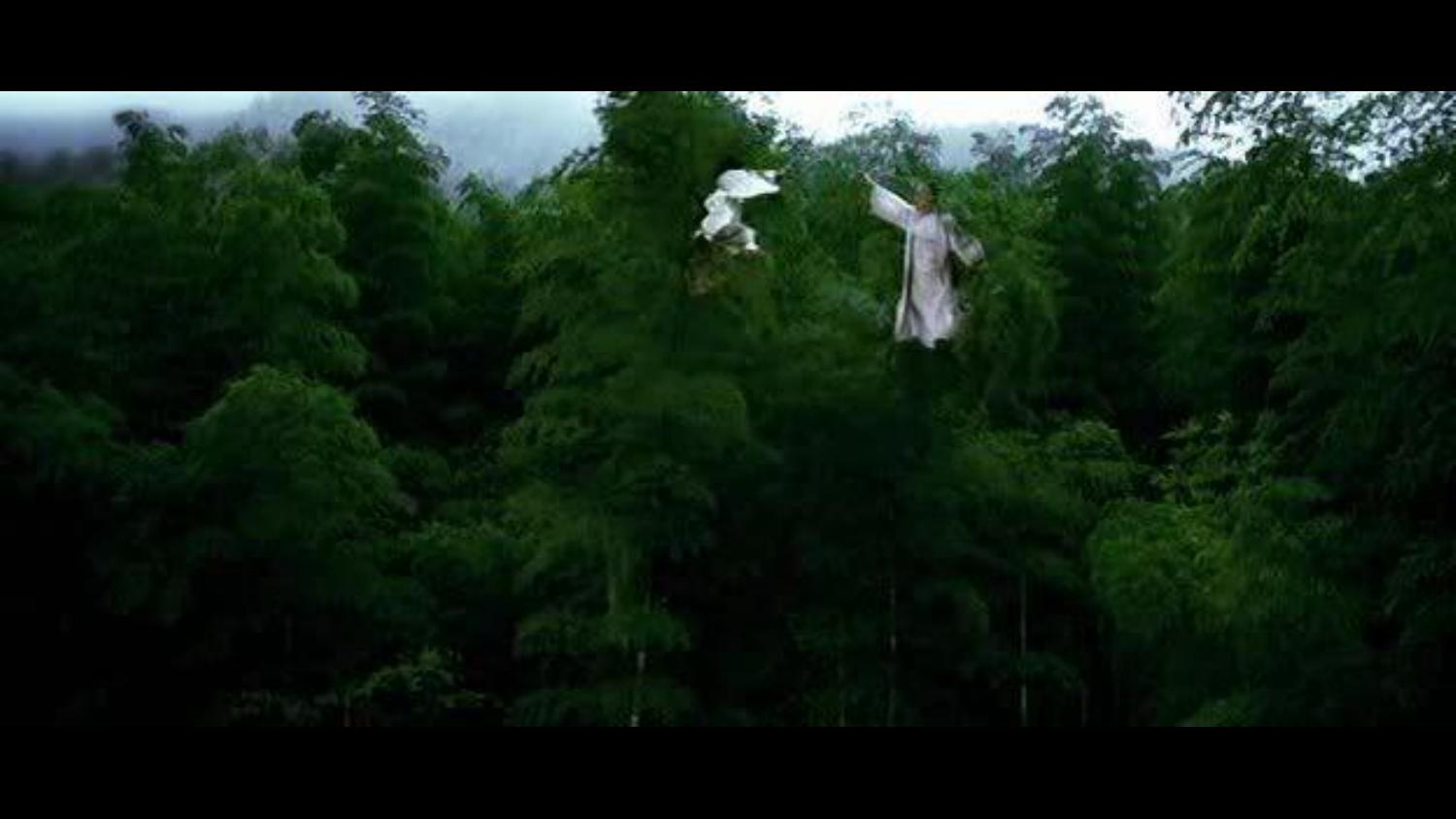
I was working as a web designer at the time, and I designed the game like I’d
design a website: start with mock-ups and concept art and then build the game
from them. I programmed for a week, using Pyglet for the first time, and found
it was just remarkably simple to use and be effective with.
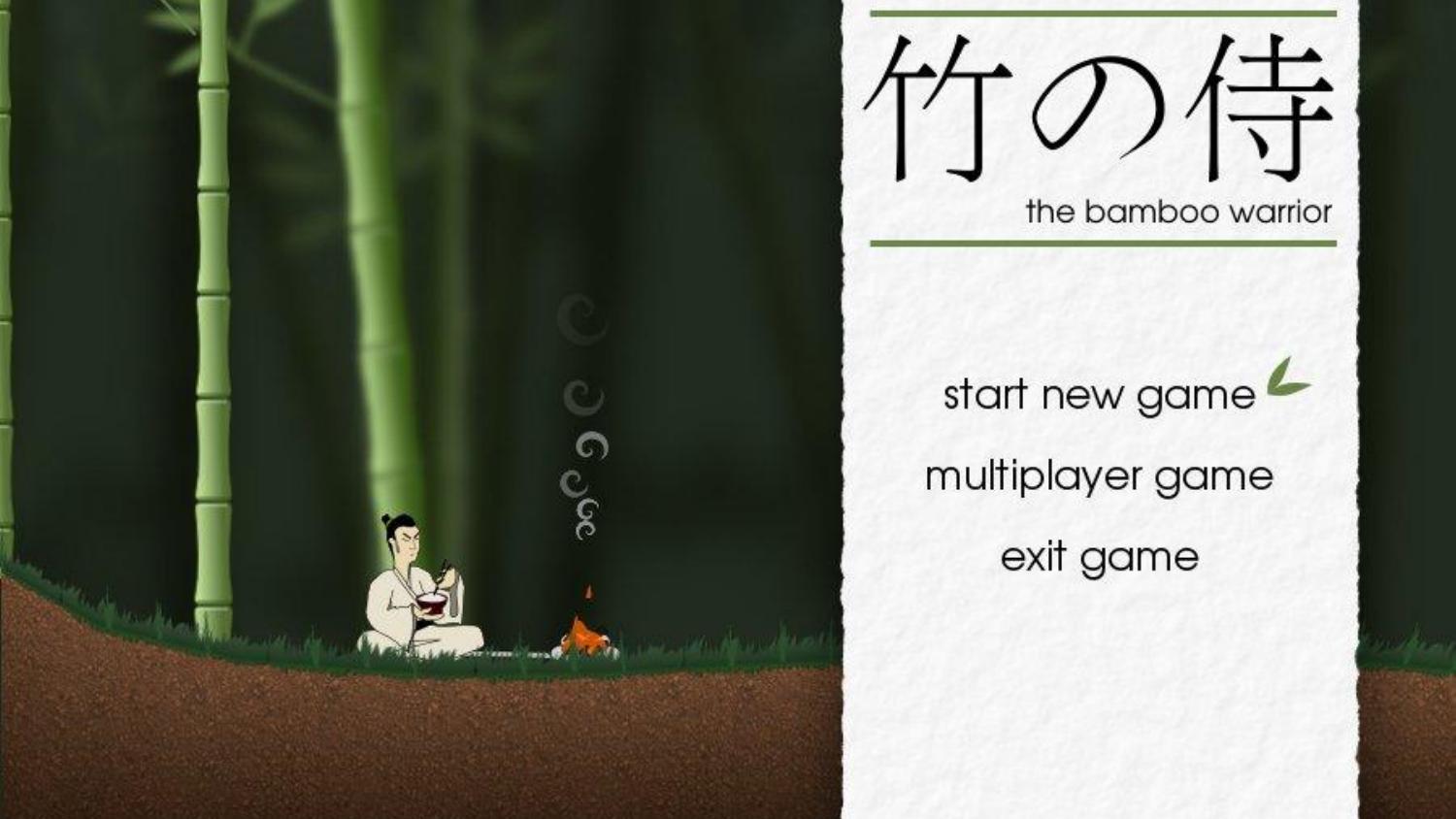
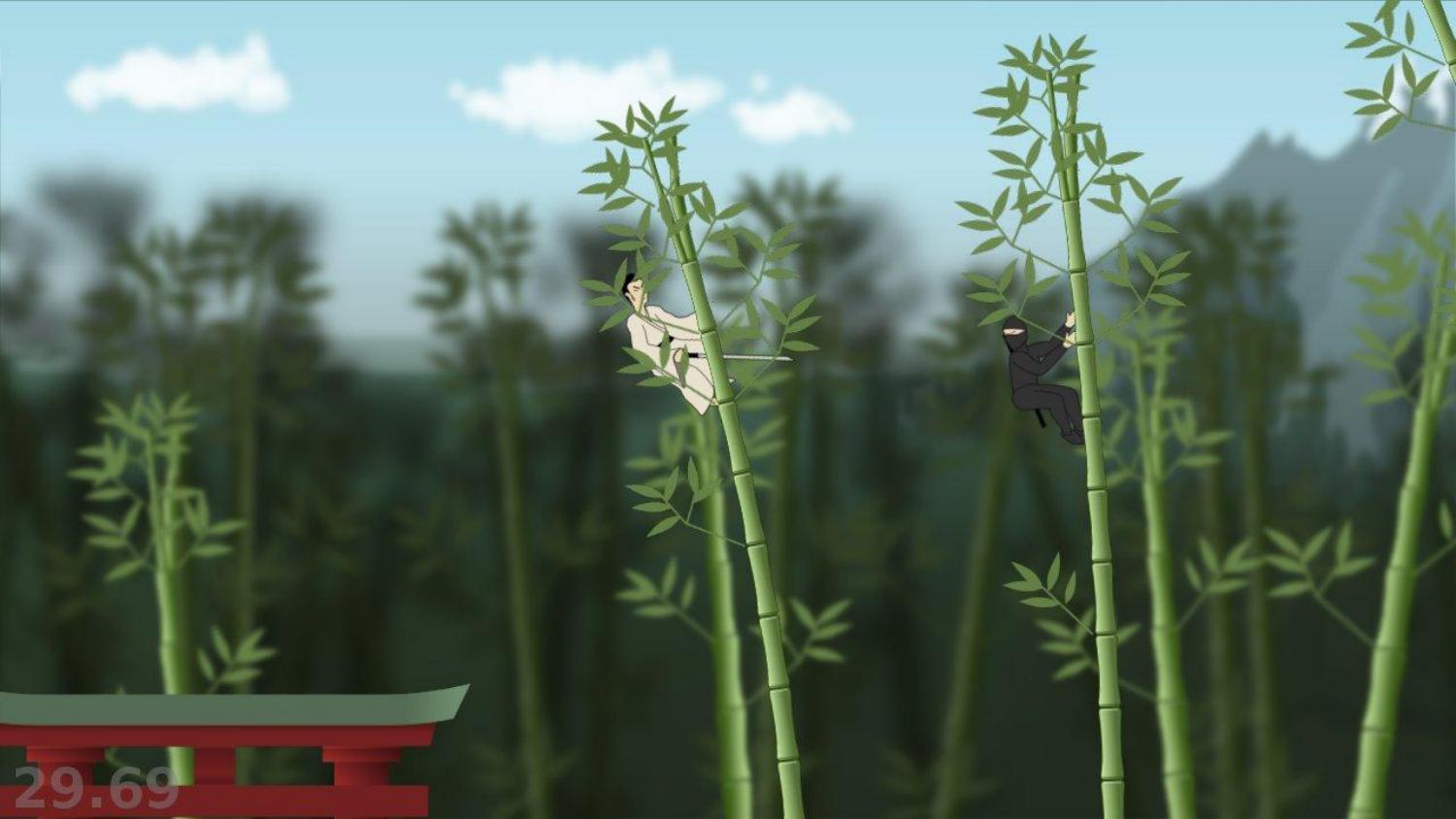
I didn’t win - though I thoroughly enjoyed the experience. It was my
most original and complicated game to date, and I’d written it in just a
week. People thought my production values were good, but the game simply
wasn’t that fun (2.9 out of 5).
Ah, fun, the elusive element, and the most important. Creating a game is
a technical challenge, and innovation is also something that but
creating something fun is something more difficult. You’re going to
spend a week programming, making choices, and creating… will it actually
be fun to play at the end?
Of all the literature I’ve read about the art of creating games that are
fun to play, and I think the best way of thinking about Fun is
maximising reward and minimising punishment.
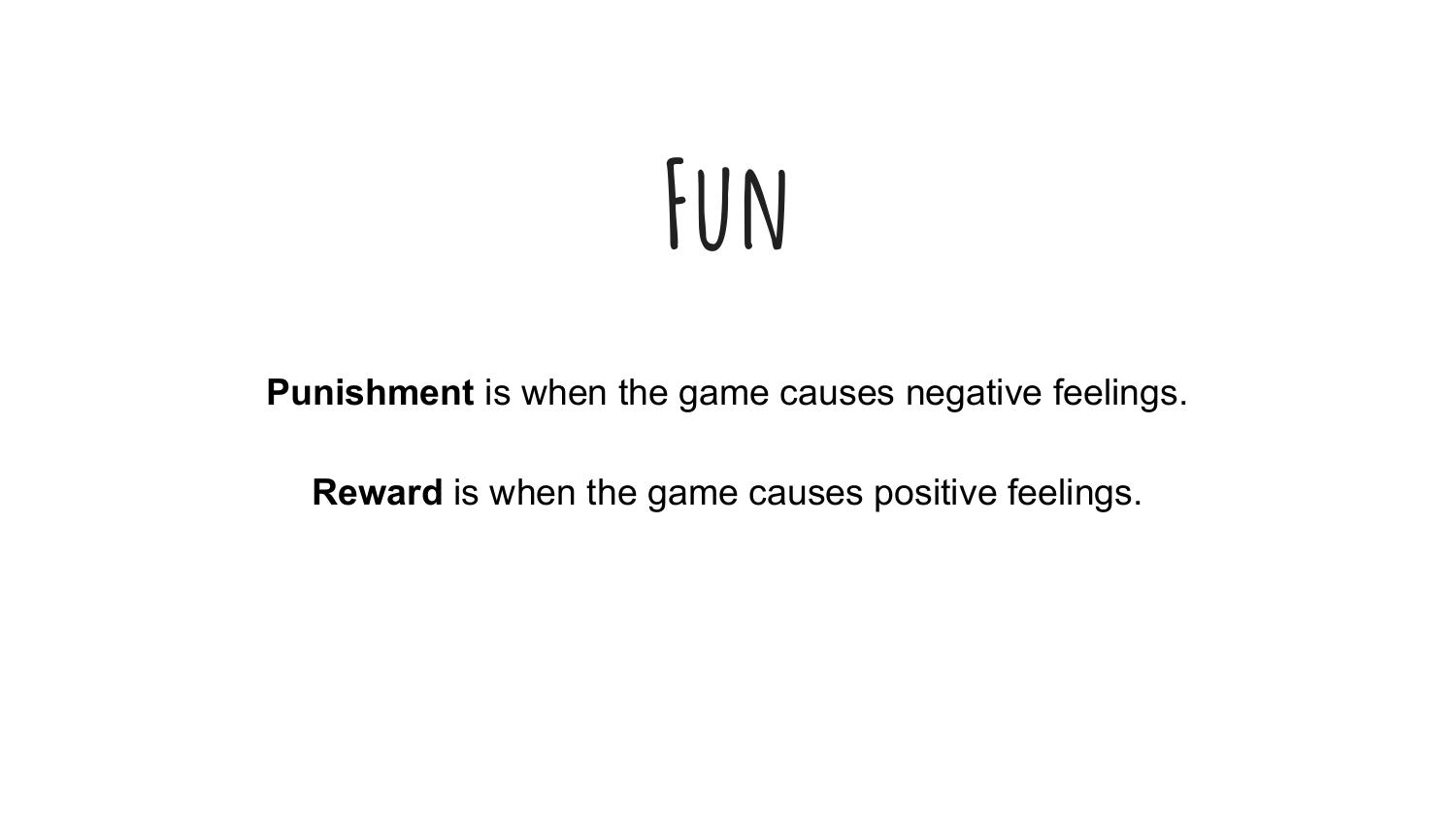
Punishment is when the game causes negative feelings. That instant
of frustration when Sonic collides with a spike and drops all his rings.
When you’re in pole position in Mario Kart when the goddamn blue shell
explodes. The boredom of a loading screen that takes 10 seconds, when
you see it 20 times an hour, is punishing. Dying in a game, and having
to repeat the last ten minutes of gameplay to get back to where you
were, is very punishing.
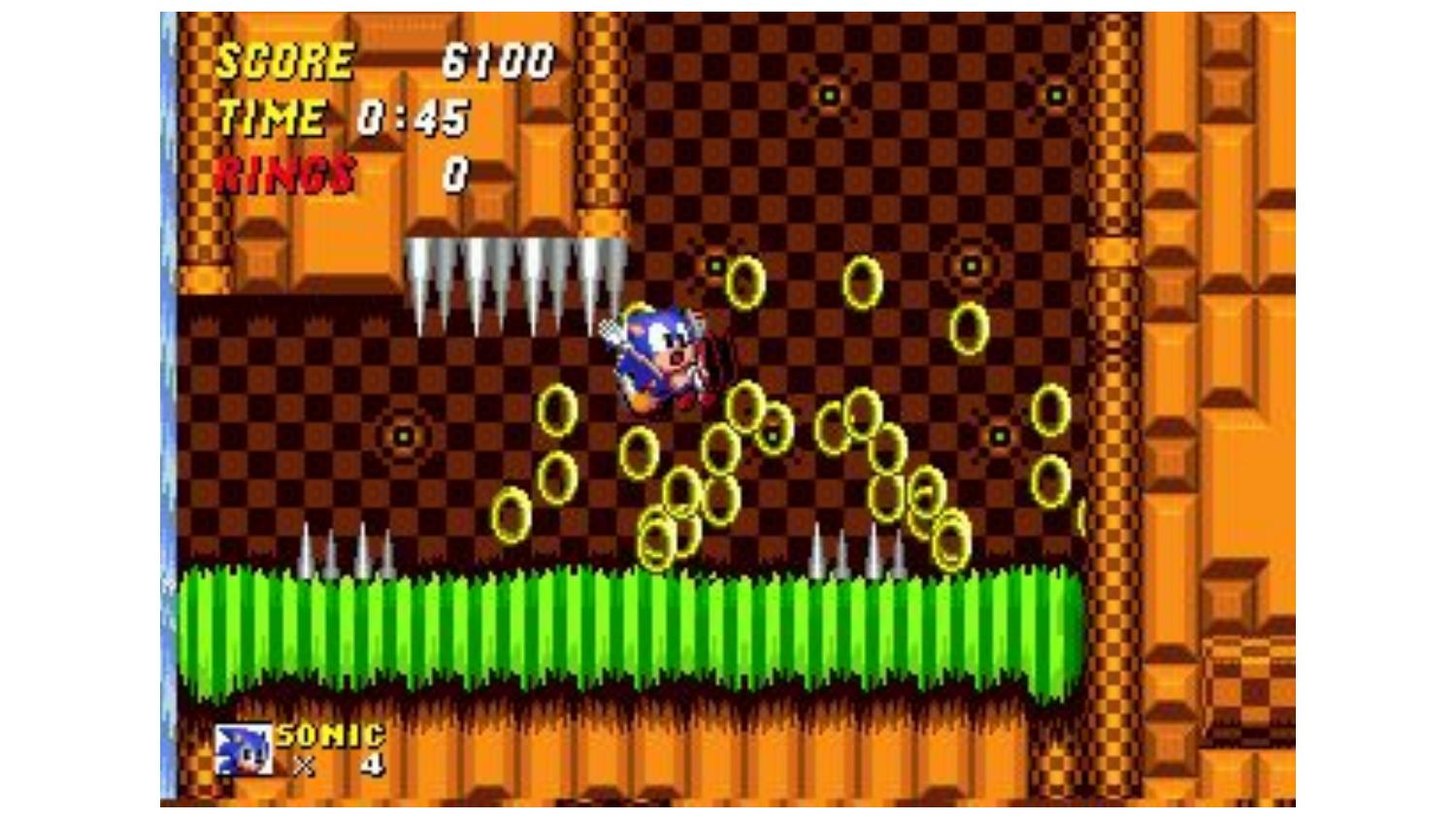
Reward is when things in the game that cause positive feelings,
however small. The surge of adrenaline when your bonus multiplier is 10x
and you’re still alive and there are a thousand enemies on screen and
you’re not sure how long you can keep this going. The discovery that you
can combine an ice potion and an arrow to create an ice arrow, and that
you can use an ice arrow to halt the waterfall so you can pass.
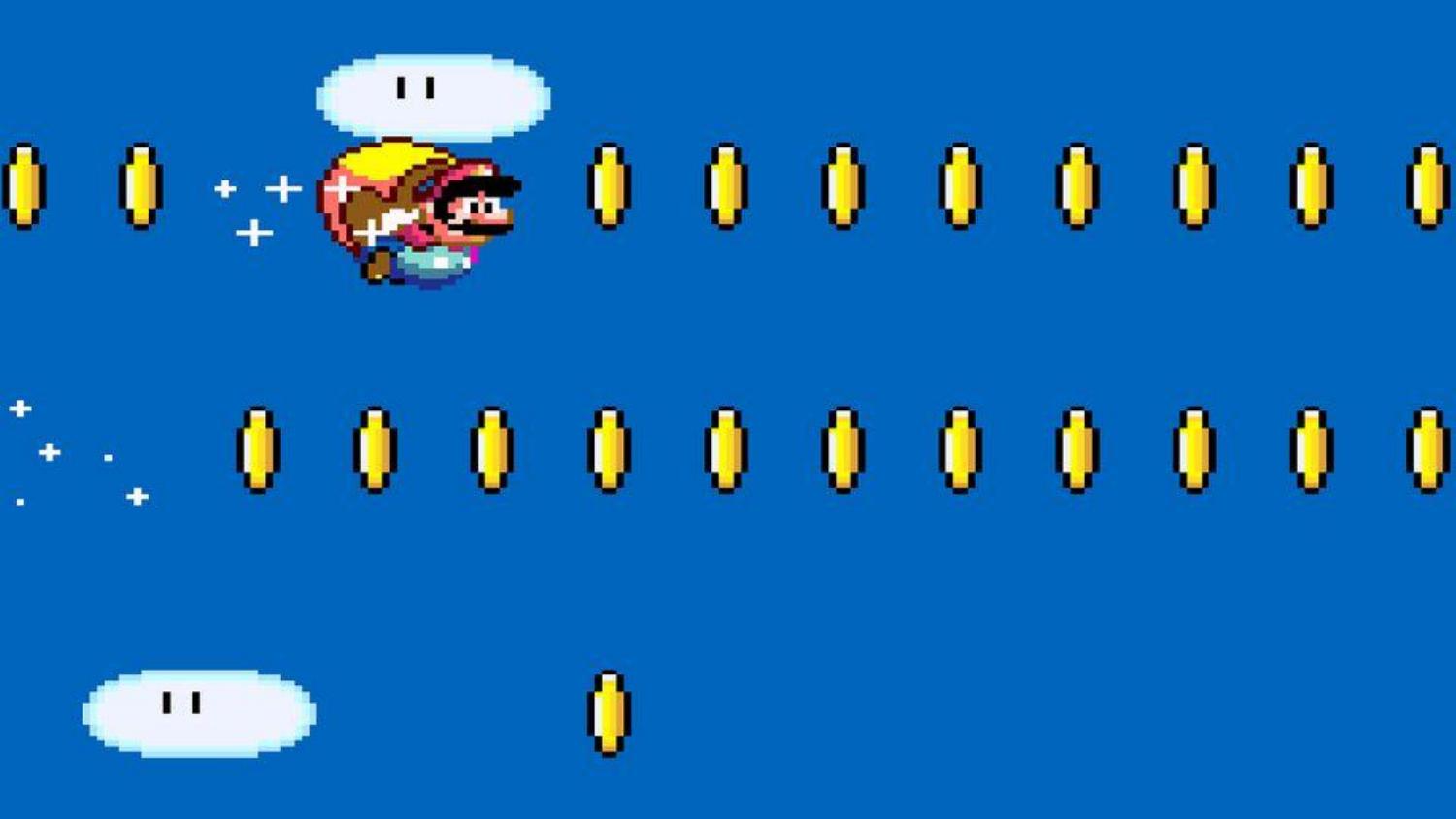
These can be the smallest things. The satisfying ‘ding’ when you collect a coin
is rewarding - that’s why in Mario people keep jumping under that block until
it spits out no more coins. The boredom of not being challenged by a puzzle is
punishing. Having to repeat the same set of clicks to open your inventory and
consume a potion is punishing.
Games are fun when they provide a constant stream of rewards and avoid
being too punishing.
If you’ve every played any of the massively commercial mobile games,
you’ll be familiar with this. Lots of the games will offer you a
constant stream of rewards - “Here, have 2 free diamonds” - with
practically no punishment (you can never lose). They dangle rewards in
front of you, withholding them just long enough to tantalise you and
make you want to pay to get them sooner.
I think this also explains why Pyweek is such fun. Python is a
low-punishment language. It doesn’t bite often. When we mess up, it
crashes with a clear traceback rather than a SIGSEGV. The libraries
don’t come with mind-bending type systems. Python is also a high-reward
language, famed for its productivity. But when we’re challenged to
unleash creativity, make something quickly, and compete with and
alongside others, the rewards are multiplied.
And occasionally, you might win.
In May 2012, my submission for Pyweek 14 - on the theme “Mad Science” -
was a physics game called Doctor Korovic’s Flying Atomic Squid. And it
won! I’m going to show you a little of it now.
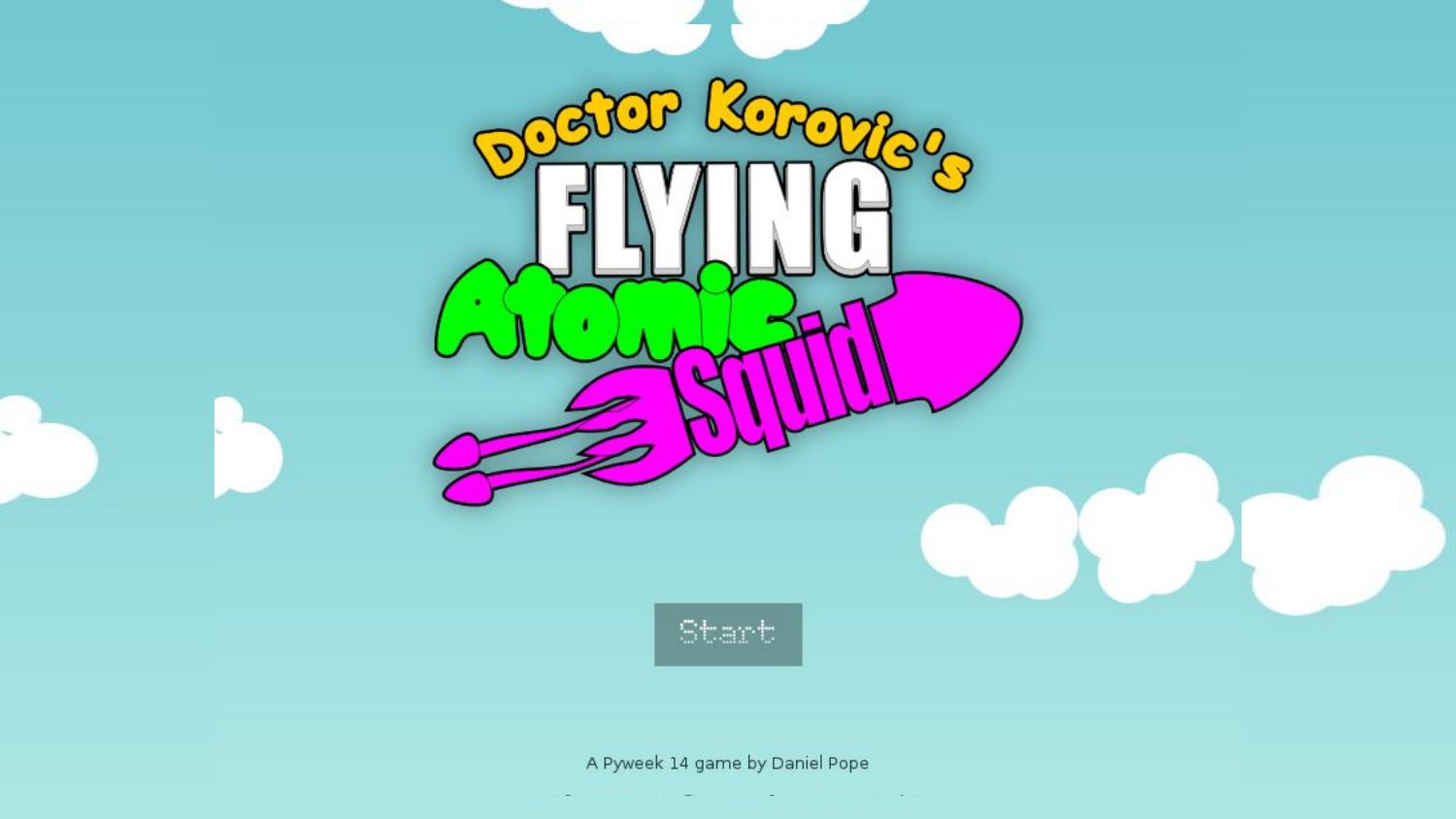
Jumping back to the story of Pyweek -- Pyweek was also conceived as a
way of energising a community to create useful tools and libraries. The
rules of Pyweek start with a mission statement:
The PyWeek challenge:
-
…
-
…
- Will hopefully increase the public body of python game tools, code
-
and expertise,
-
Will let a lot of people actually finish a game, and
-
May inspire new projects (with ready made teams!)
Because of the “from scratch” rule, from the outset people would create
and publish libraries from their Pyweek games to avoid having to write
the libraries again.
The second Pyweek competition ran in March 2006. The individual
competition was won by Alex Holkner, with a game called Nelly’s Rooftop
Garden, and it is still the highest-scoring Pyweek game of all time.
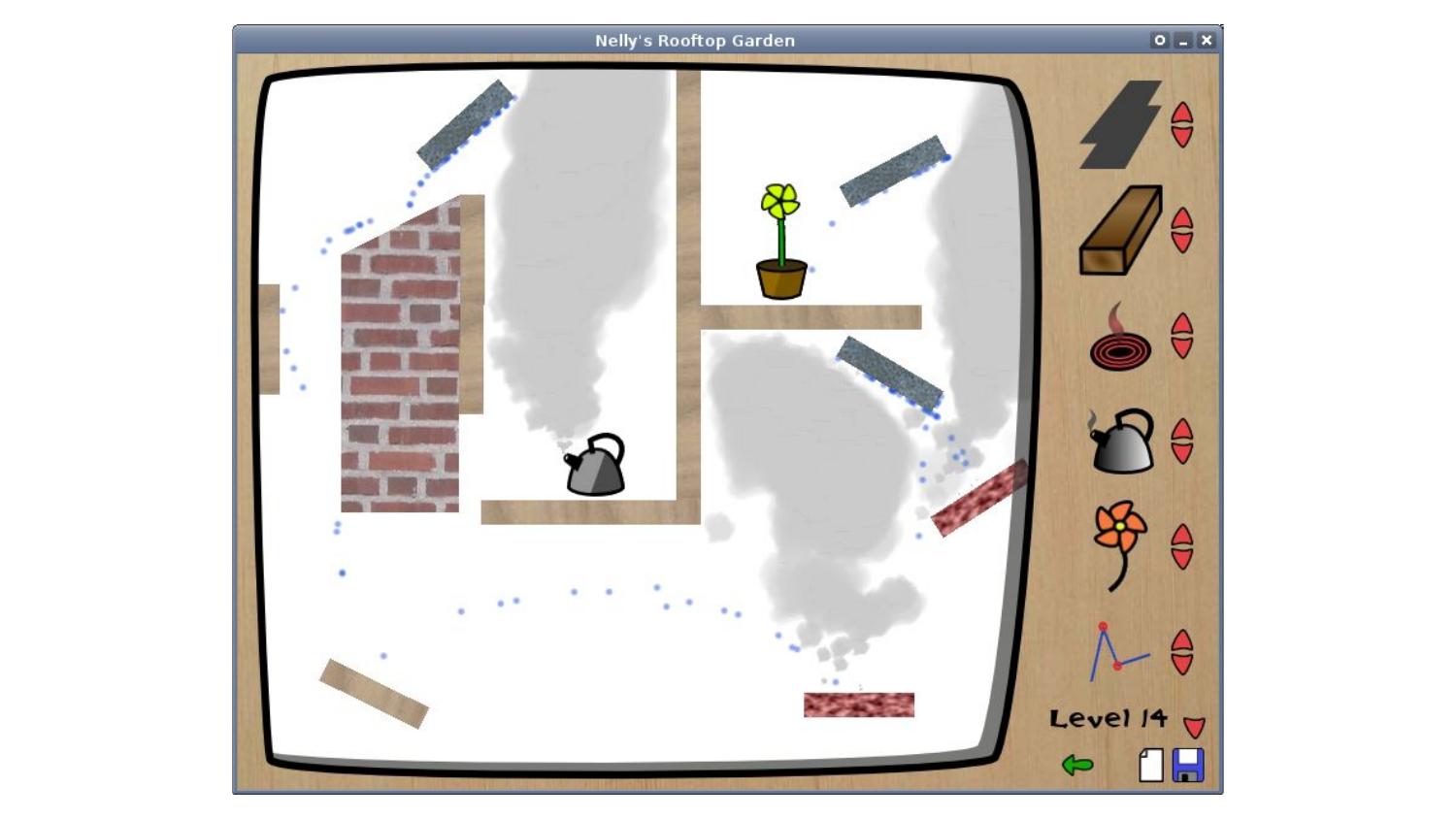
Later that year, Alex started a Google Summer of Code project to create
a clone of Pygame using the ctypes library, which having been available
for a few years, was due for inclusion in the Python standard library in
Python 2.5 that autumn. His mentor was Peter Shinners, the Pygame
author.
He quickly got good at this. In a few short weeks he had a working
implementation of Pygame with ctypes, as well as a lower-level
SDL-ctypes. And then he started looking at PyOpenGL, which had some gaps
in API coverage. He started ctypes bindings to fill those gaps - and
quickly realised he could produce complete ctypes bindings for OpenGL
relatively quickly. The project was named pyglet.
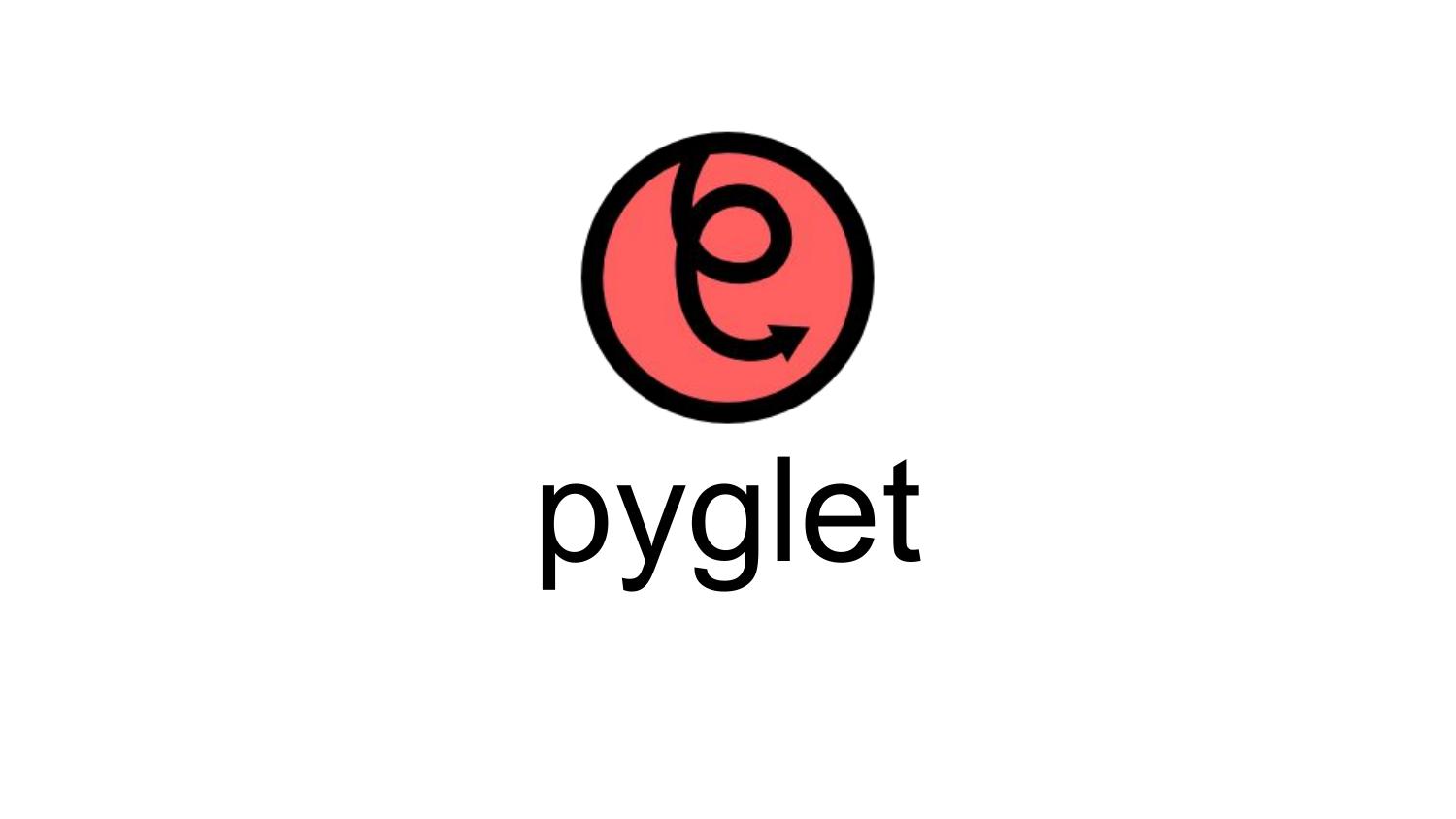
At this point the ever active Richard Jones pitched in and together
Richard and Alex forged Pyglet into a full cross-platform,
dependency-free games library. Alex pulled text rendering from Nelly’s
Rooftop Garden. Then Pyglet gained a featureful sprite library, with
support for rotation and scaling of sprites, as well as colours and
transparency, and a native sound library called AVBin.
Pyglet is easy to use for beginners, while exposing the underlying
OpenGL primitives to more experienced users. Its sprite and text
libraries can be wrapped up with OpenGL state changes to achieve complex
effects.
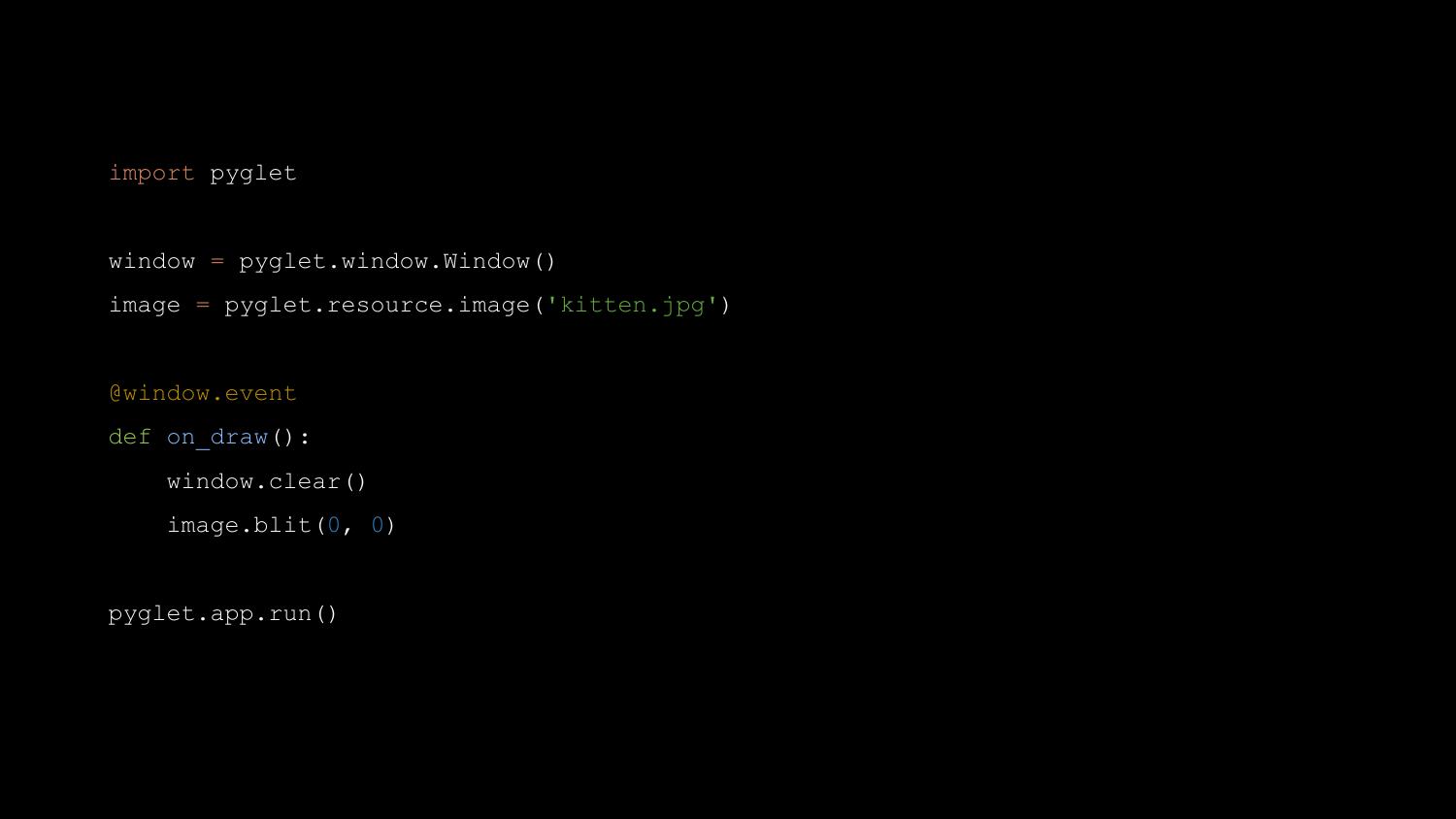
Pyglet is a great library, and it is what I used in the majority of my
Pyweek entries, including Doctor Korovic’s Flying Atomic Squid. It has
more graphics features than Pygame, and is probably as easy or easier
for the basics - but Pyglet does offer the full power of OpenGL at the
cost of complexity.
A year and a half after Pyglet was released, at PyCamp in Los Cocos,
near Córdoba in Argentina, Ricardo Quesada and others started the “Los
Cocos” Python game engine, a higher-level engine based on Pyglet. It was
soon renamed Cocos2d. Ricardo later ported it to iPhone, where it became
wildly popular. Many App Store number one games have been written with
it. There are over 100 books about Cocos2D programming.
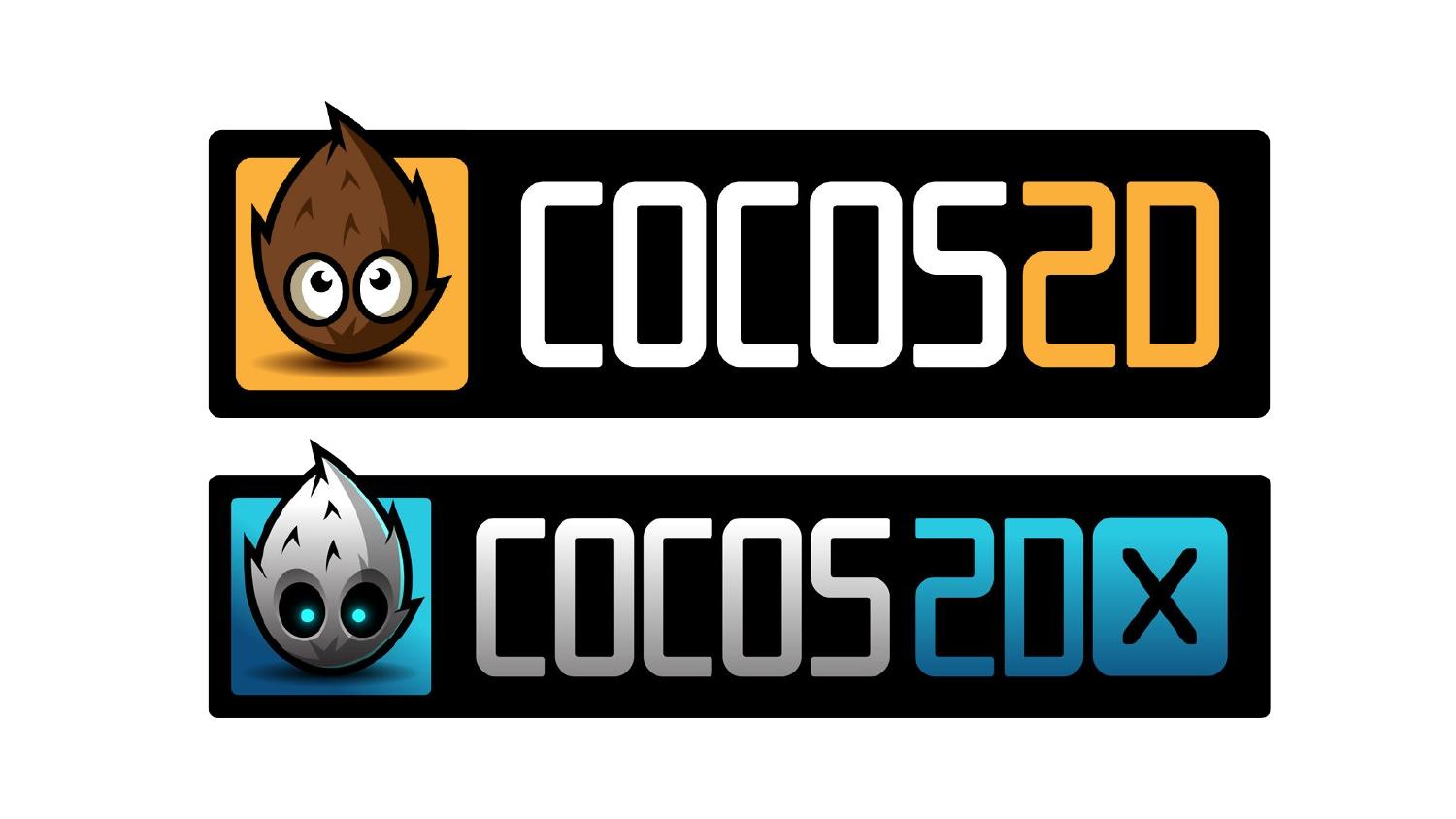
So Pyweek is a successful incubator for technology.
Let me pick up another thread of history now - this Education Track at
Pycon UK. Pycon UK’s education track was set up by Nicholas Tollervey -
who has keynoted here previously - and who is himself a former teacher.
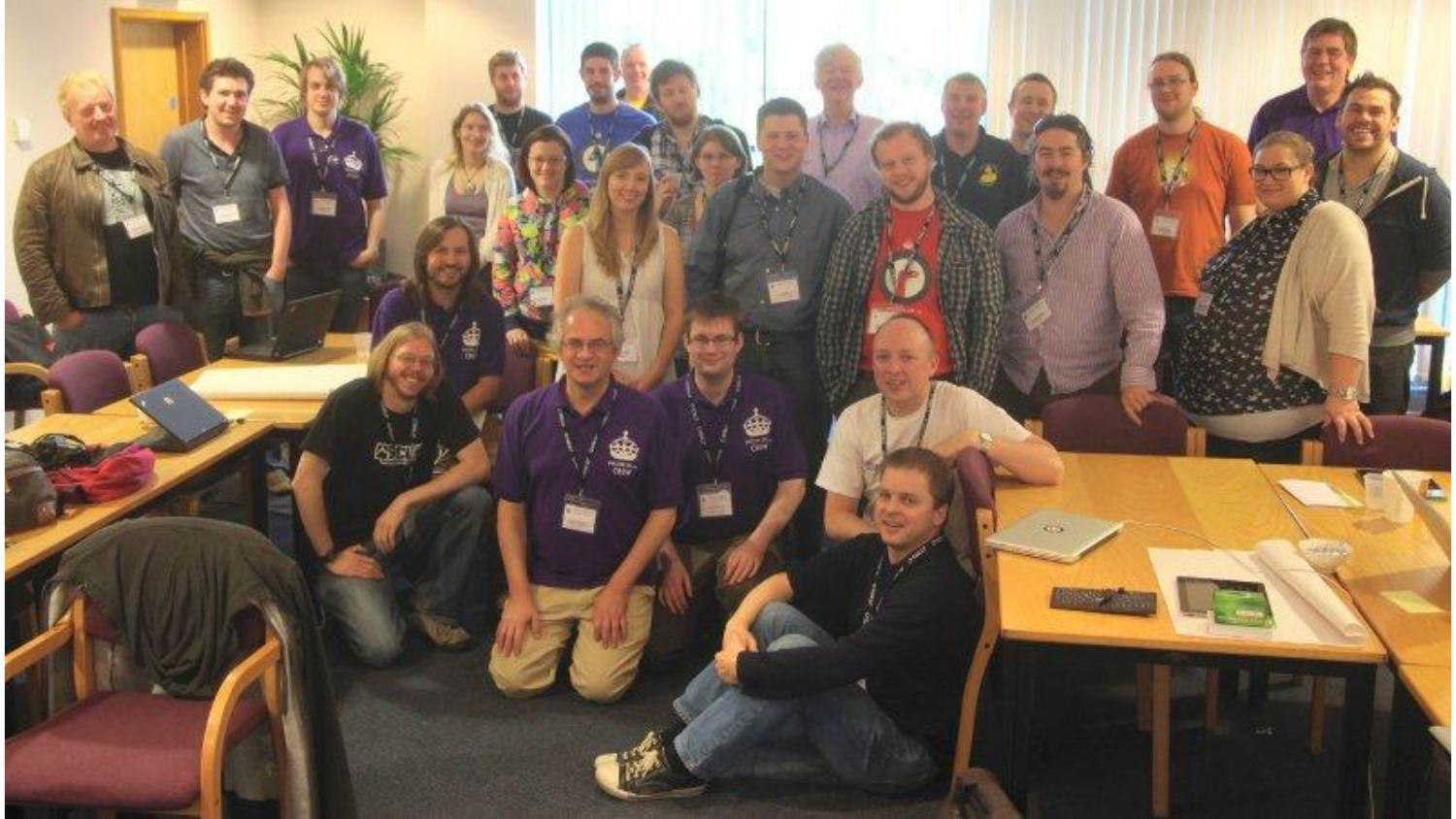
The very first education track, in 2012, invited teachers to attend
Pycon UK for the first time. The teachers were “shared out” between
Python programmers, and each group was challenged to come up with course
material. I was there that first time, fresh from my Pyweek win, and I
was sorted into a group with a teacher named Ben Smith. Ben is a
teacher, and was keen to see what I could come up with for teaching
programming to kids through the medium of games.
I spent five minutes or so writing a basic Pygame game, which looks like
this:
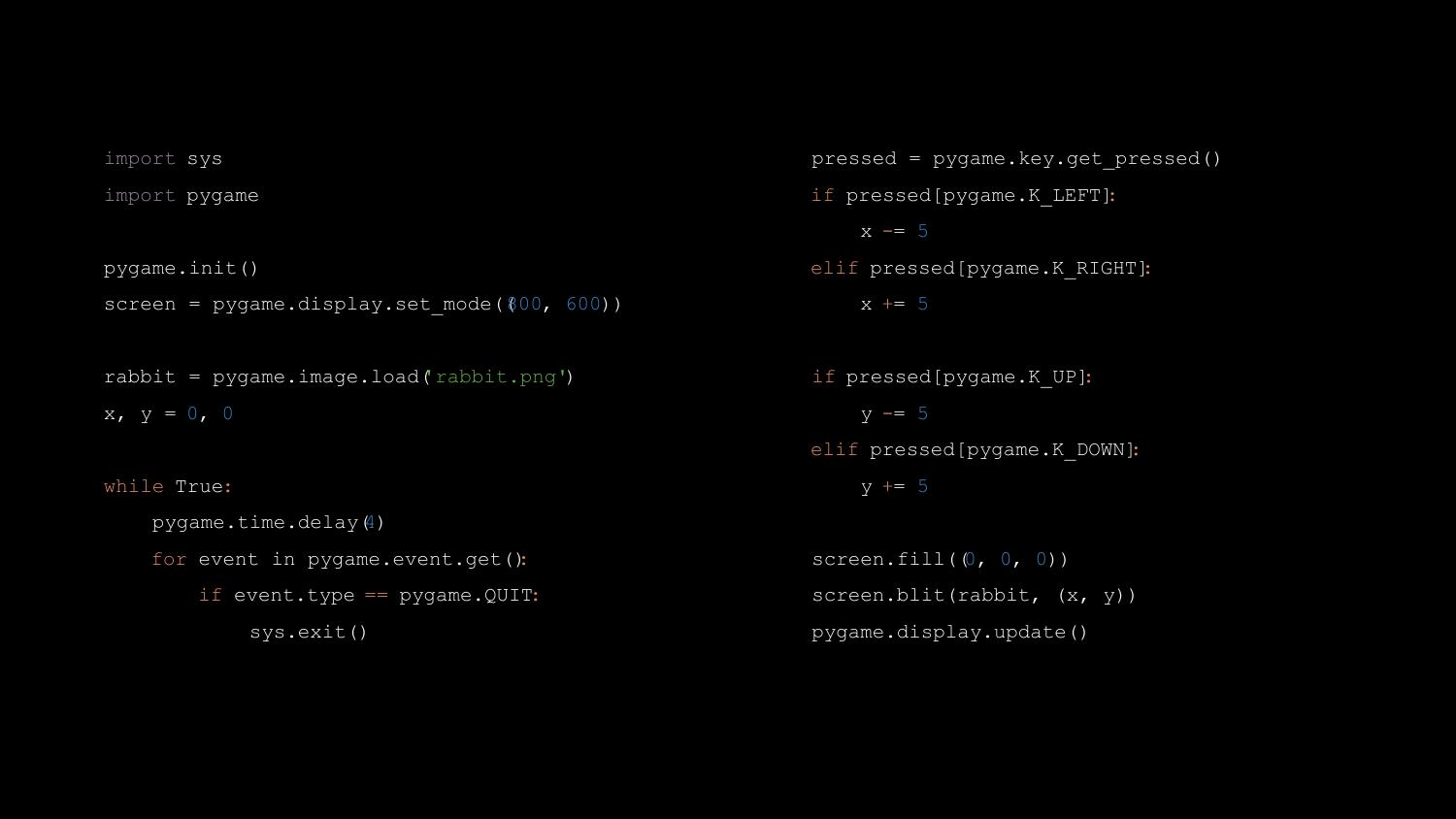
Looks simple, right? But Ben told me the first moment he saw it, “I
can’t teach that.” The problem was that it was just too many lines of
code. In a 40-minute Computing lesson, he would have some students race
through it in five minutes, while others would be struggling to type the
first “import pygame” line correctly. I was a bit taken aback, to be
honest. It needs to be simpler than that!? But, as he explained, he
needed to be able to feed them a sequence of tasks that were small
enough that everyone could catch up, before moving on.
The education track has run every year since 2012, and I’ve attended it
every time. As well as the teacher’s day, it now also has a “kids day”
where kids can come and learn to do fun things with Python. A few years
ago, I was working for Bank of America Merrill Lynch, and I helped
arrange for the bank to sponsor the education track, which it has done
since 2014. The sponsorship money helps, for example, to pay for supply
teachers so that the regular teachers can come.
Teachers in the UK are facing an immense challenge in teaching a modern
computing curriculum. Many teachers don’t have programming skills
themselves. Most schools lack resources to train their teachers, procure
hardware, or install and configure the software needed to teach
programming skills.
Still, through Pycon UK I’ve met many amazing teachers who overcome all
this with ingenuity and enthusiasm. They make programming fun.
In October 2014, after a few attempts to take the Pyweek team entry
title had failed, I won the Pyweek solo competition again, with a game
called Legend of Goblit. The theme was “One Room”, and Goblit is a
LucasArts-style adventure game set in a single room. It’s actually a
sort of “adventure stage play”, with lots of scripted sequences, pausing
for interactive puzzles. Let me show it to you now.
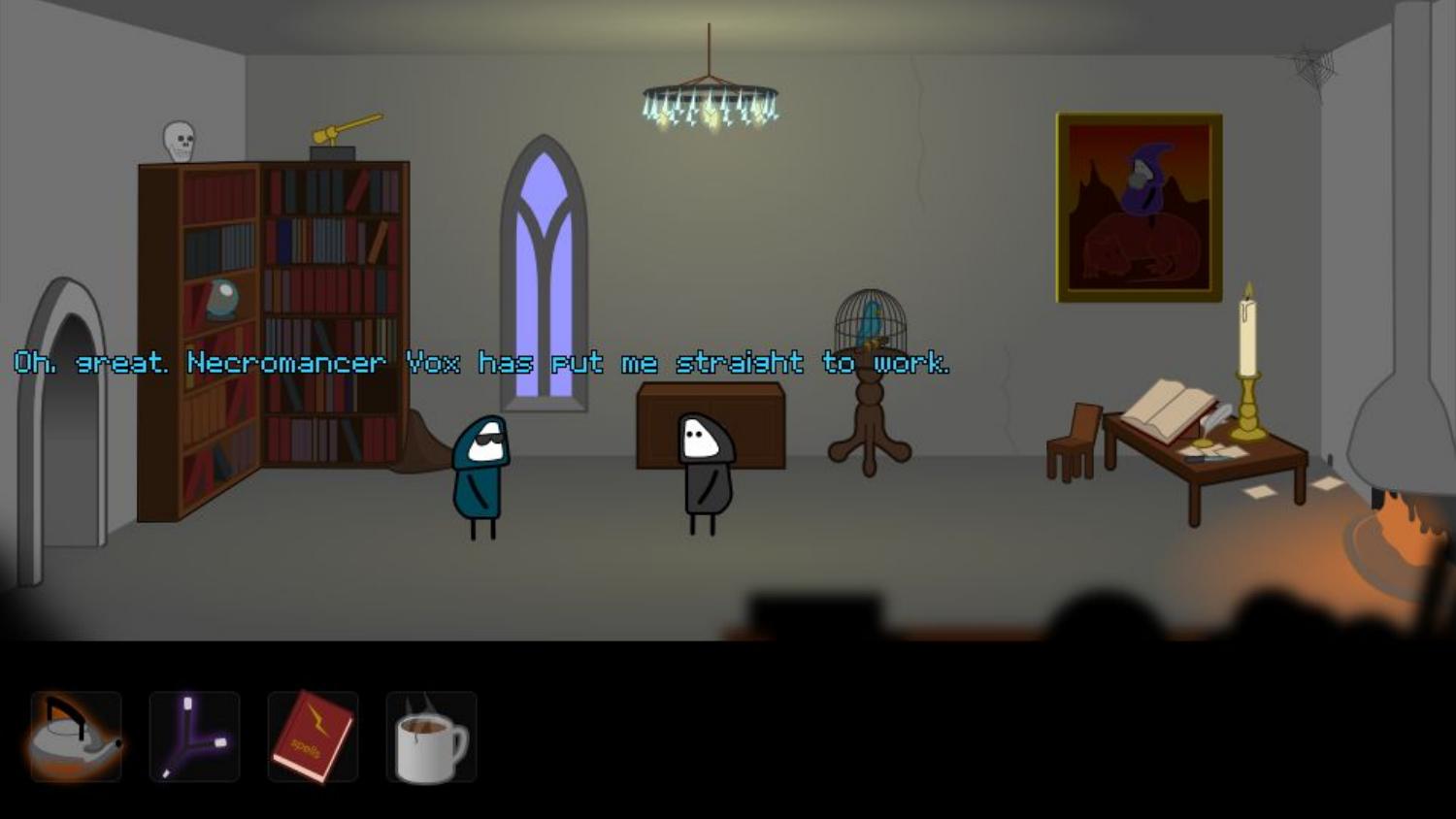
Goblit was probably not the most innovative game that time - but it
was the most fun. In fact it is the third most fun Pyweek game of all
time, behind Nelly’s Rooftop Garden (second) and Mortimer the
Lepidoptorist (first).
Legend of Goblit was my first Pyweek entry in a few years that was
written with Pygame. As I wrote it, I had something at the back of my
mind - I thought back to that education track, to what Ben Smith had
said about Pygame being too complicated. Part of the code of Legend of
Goblit was a small Pygame-based game engine that was intended to be
accessible to complete beginners.
Well, as usual, that sat there for six months, until Pycon 2015, in
Montreal, Canada. On the first day of the sprints, I was sitting with
Richard Jones, and I told him that I was proposing to sprint on writing
a Pygame-based game engine for education. Well, as you’ll understand by
now, when Richard is into an idea he’s into it - and he joined me to
sprint on the new library, which I named “Pygame Zero”. This is us
working together on the first cut of Pygame Zero.
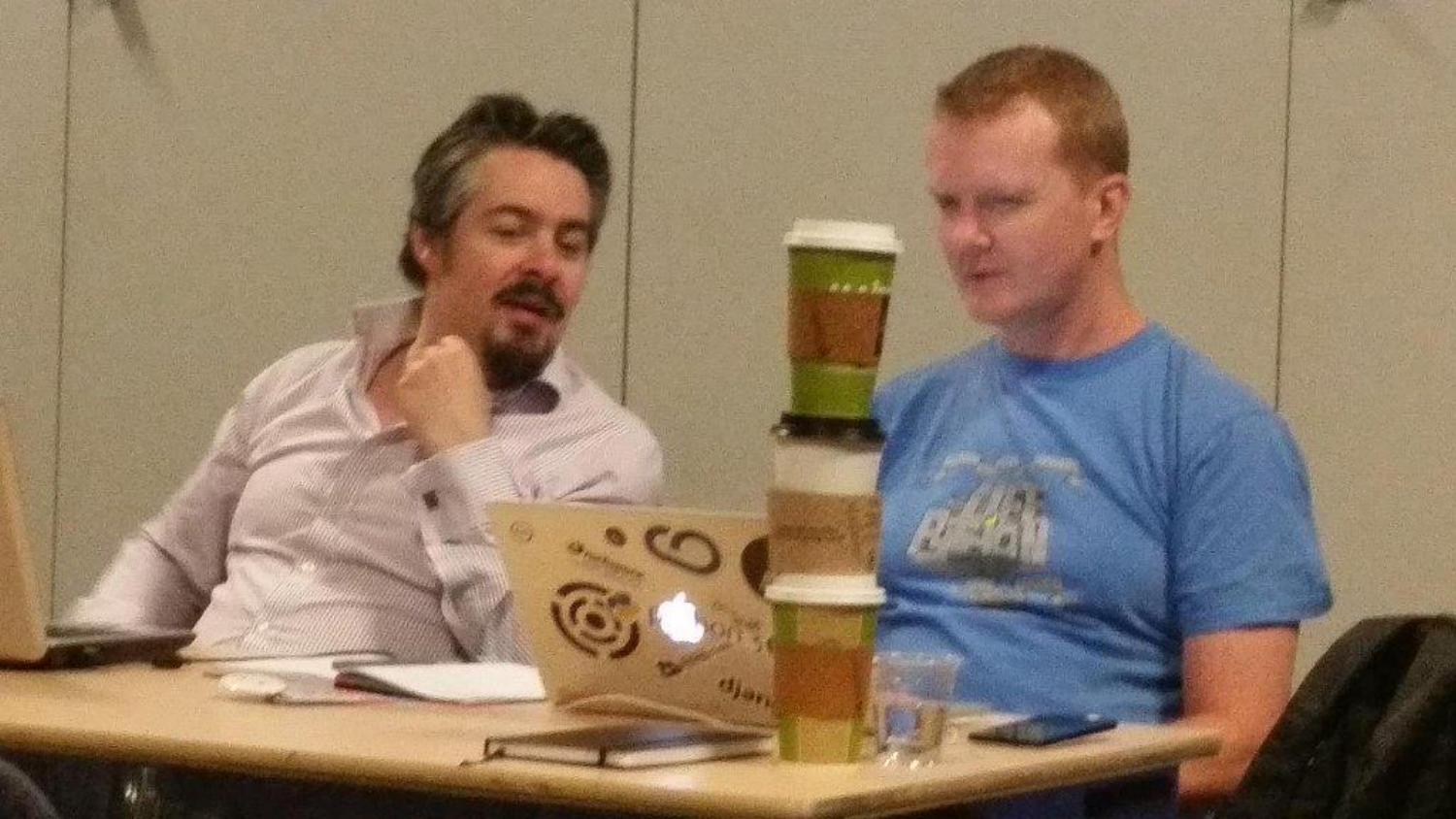
Pygame Zero is a zero-boilerplate game engine for Python. What do I mean
by zero-boilerplate? An empty file is a working Pygame Zero program.
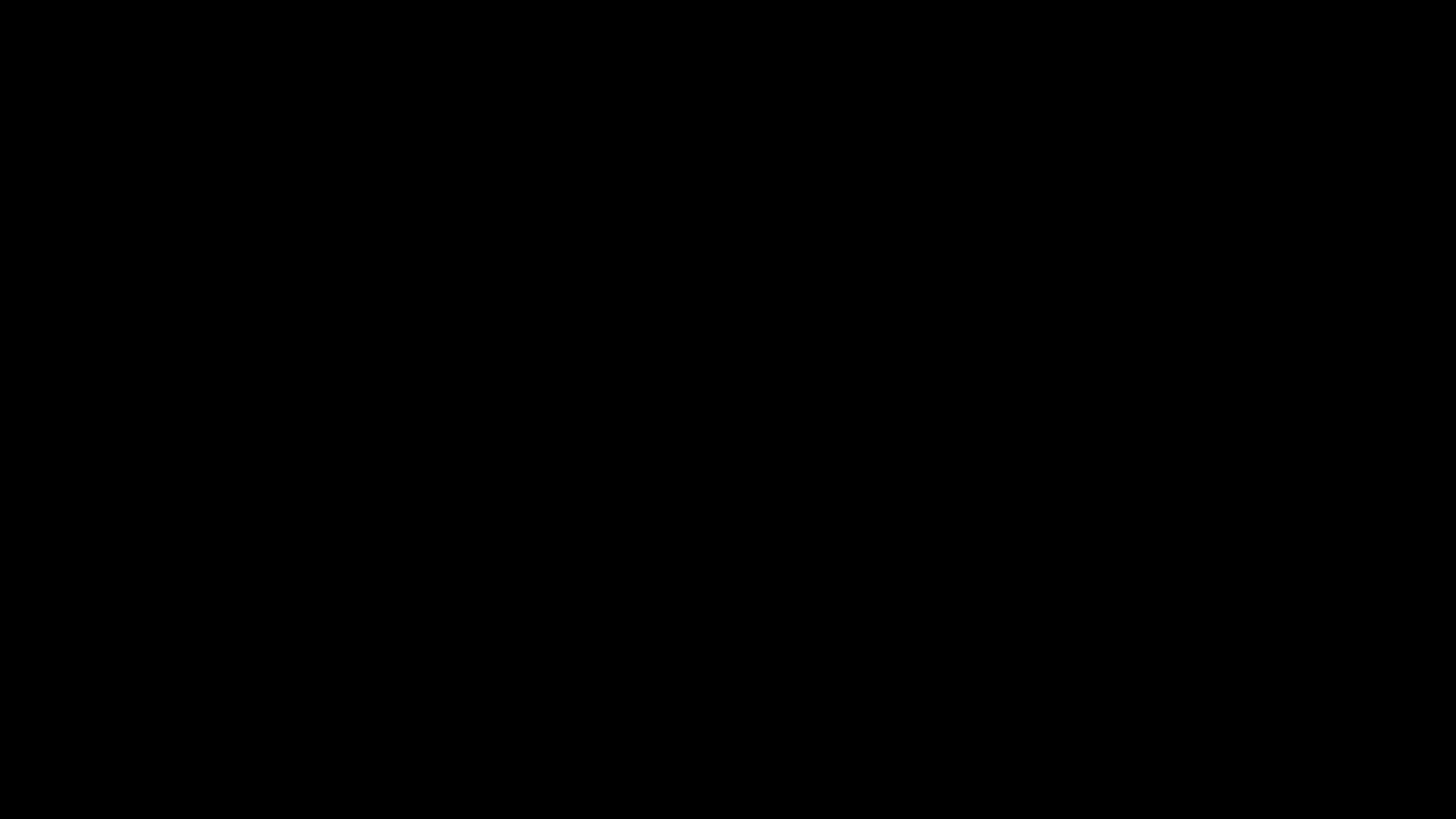
We don’t run this with the standard interpreter; we run it with pgzrun.
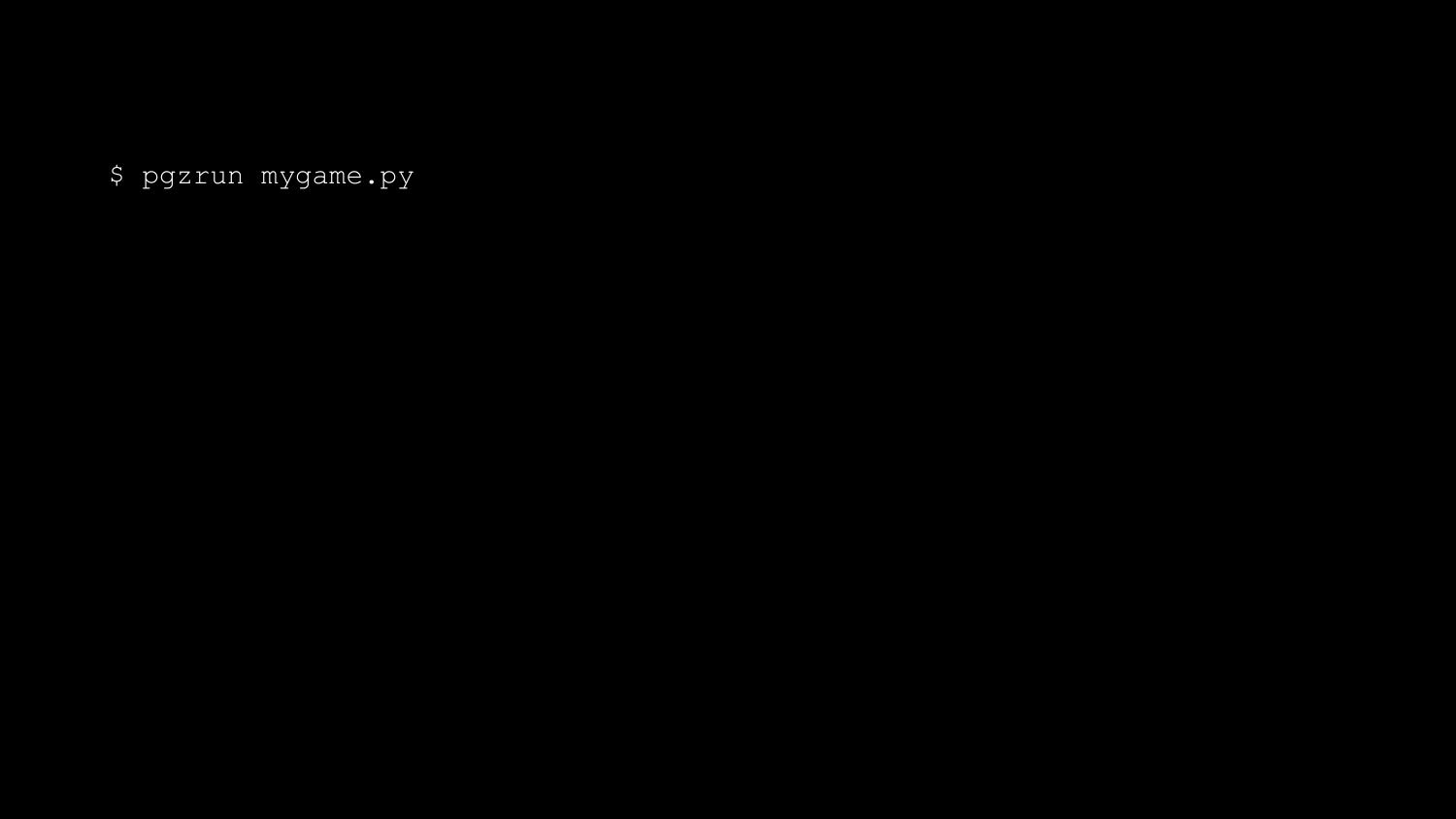
Next can add a draw() function.
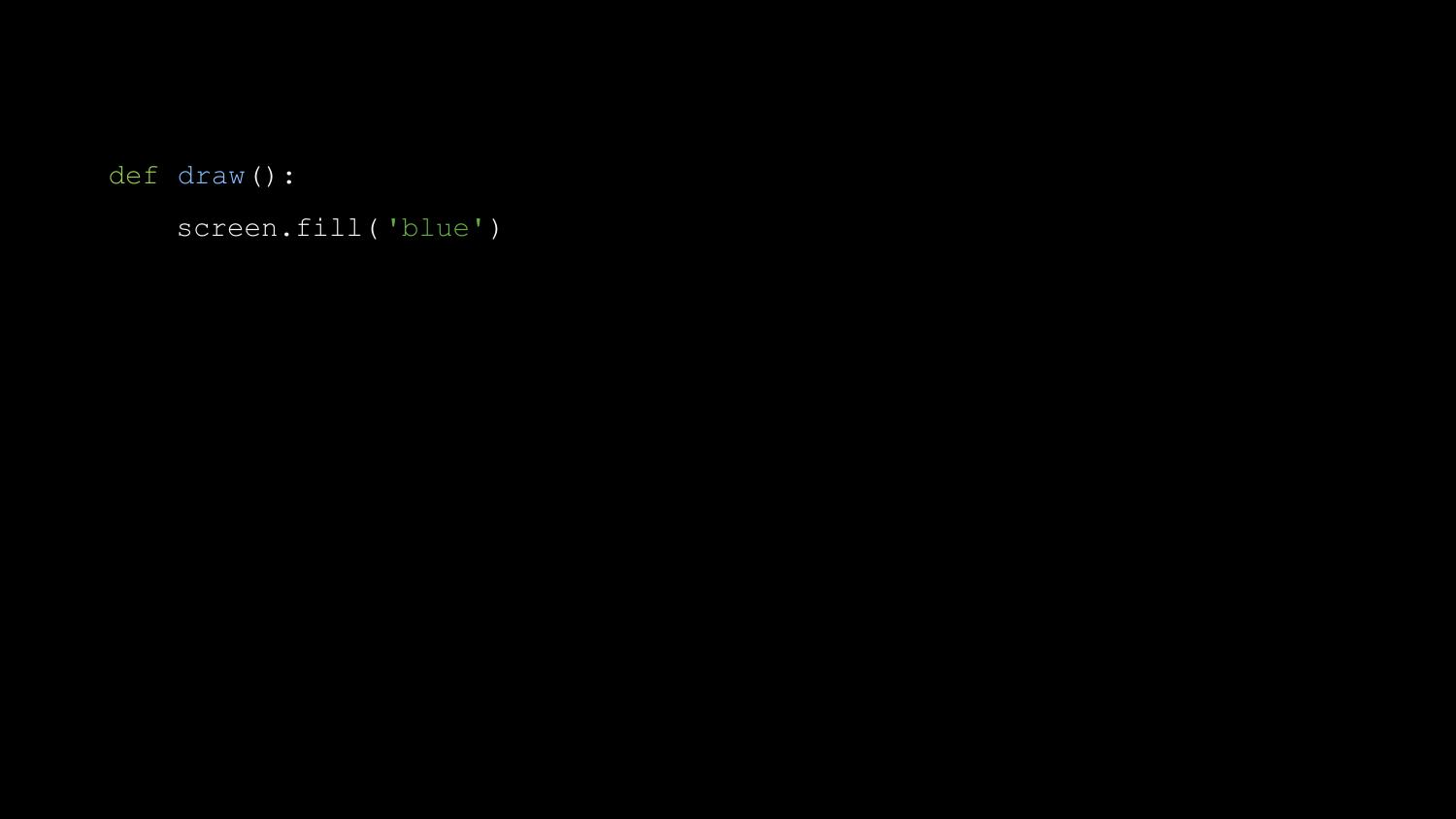
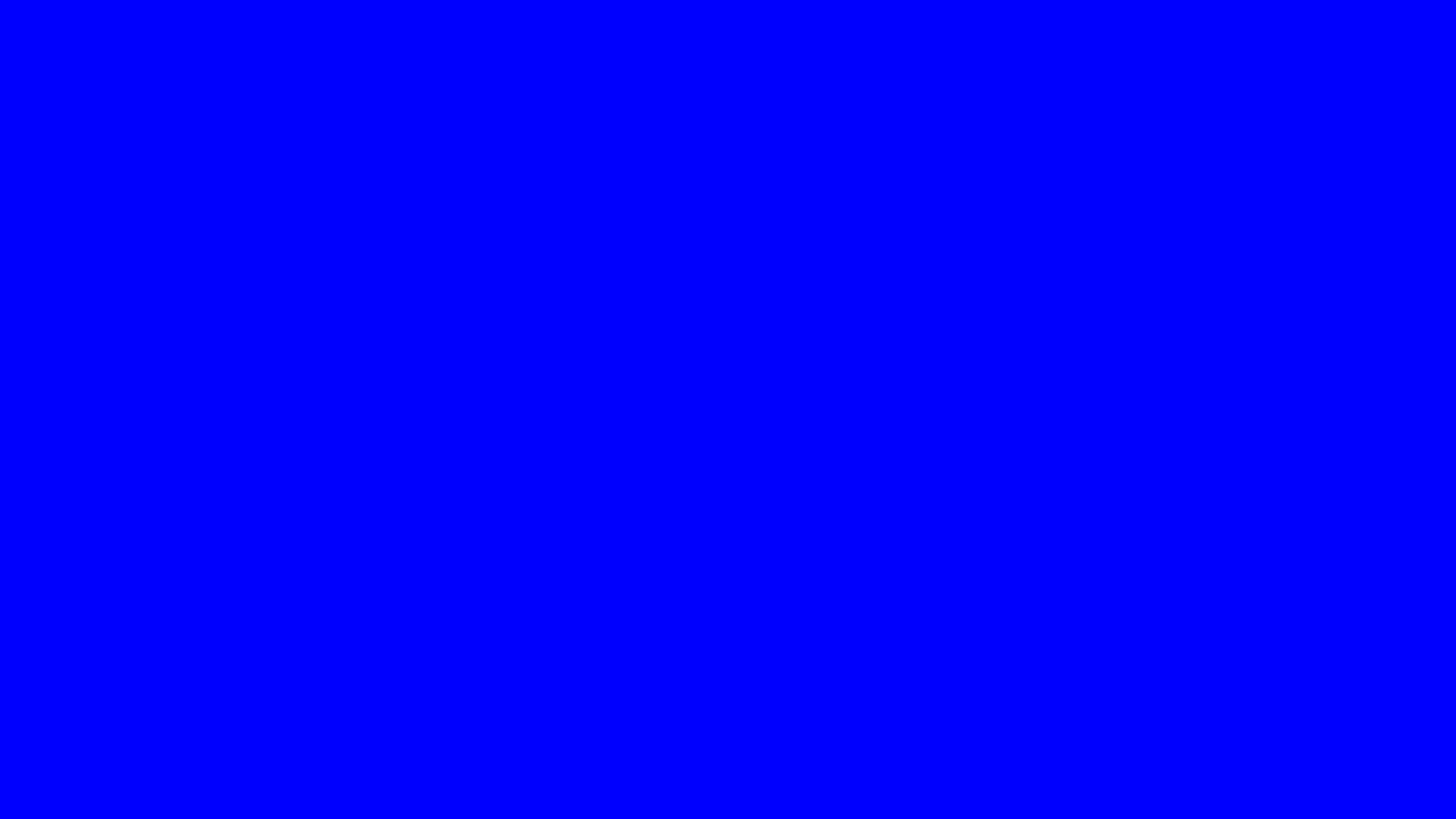
You might use this to draw an Actor - in this case a rabbit sprite
loaded from a file called ‘rabbit.png’.
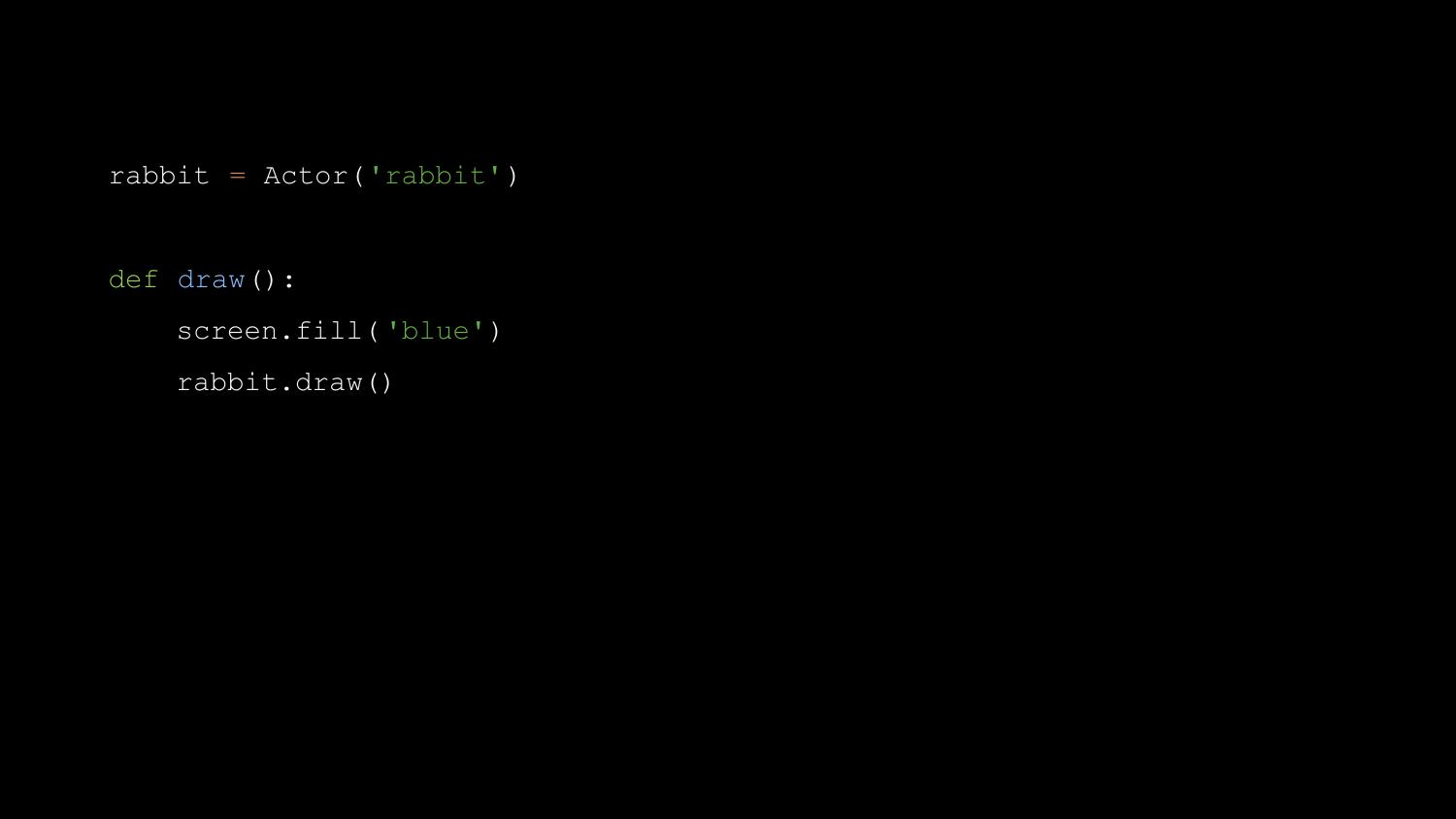
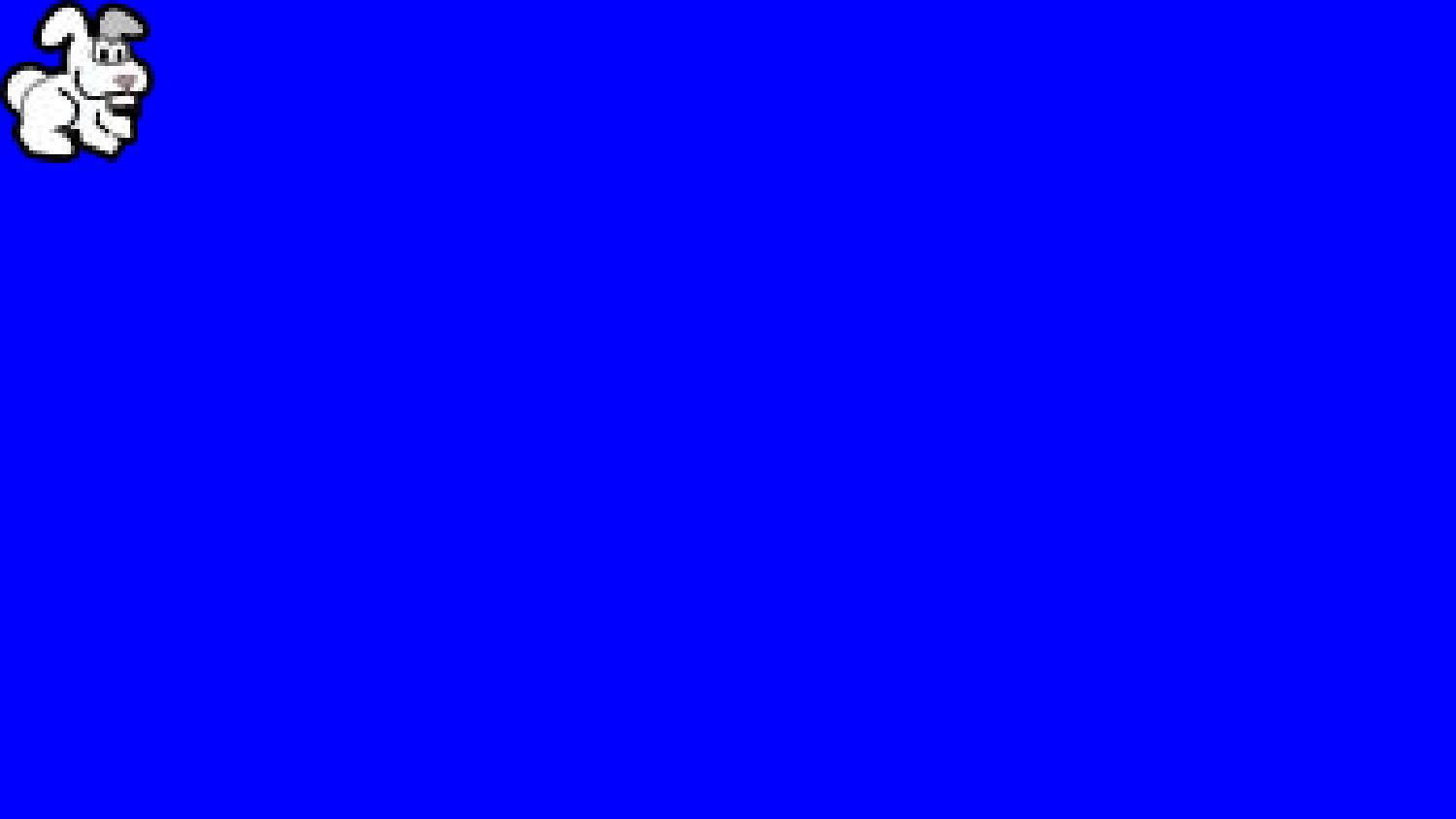
Then we can write an on_key_down() function, to handle input.
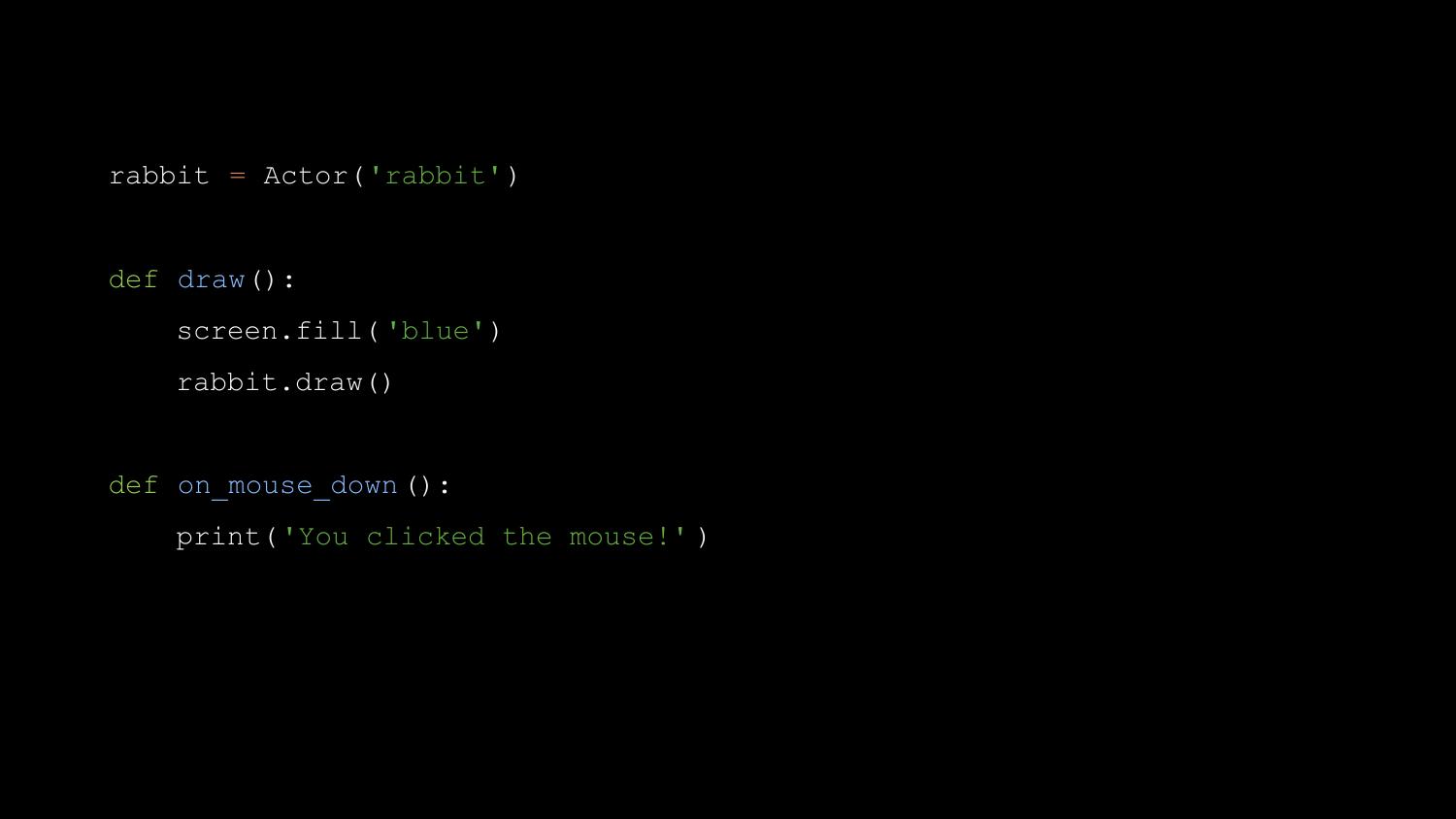
And if we add a button parameter to the on_key_down() function, this
value will be passed into the function.
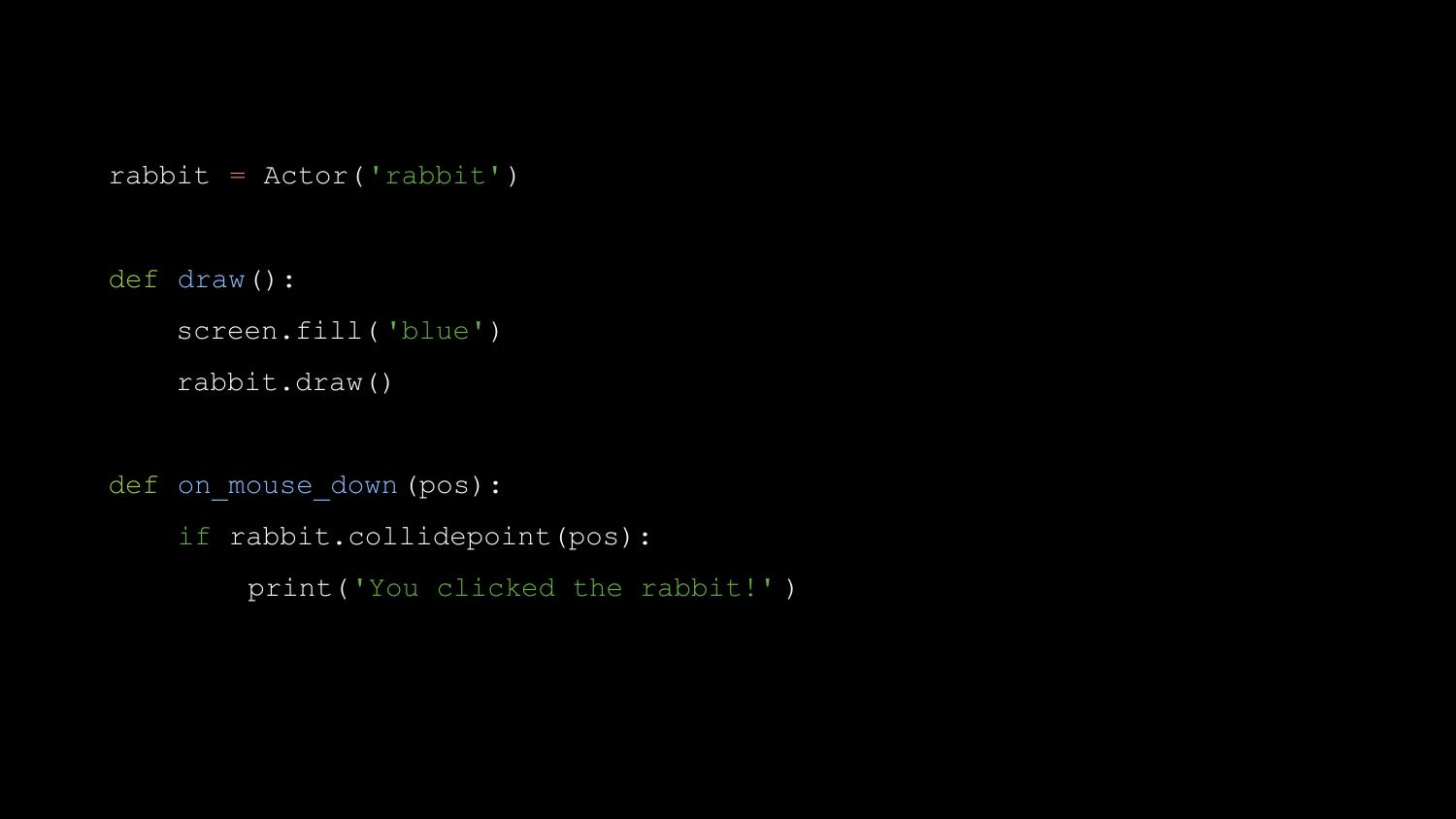
At each stage, we’re adding just a couple of lines at a time, and
getting immediate results. If you think back to Ben Smith’s criticism of
Pygame, to the problem of feeding his class a bite-size piece of work
that would let groups all catch up before moving on, Pygame Zero solves
it.
We use a little bit of magic - some extra builtins, some metaprogramming
- so that we don’t have to teach import statements before we teach how
to define and call a function.
Let me show you something else. Another teacher I met at Pycon UK was
writing his first Pygame Zero program, and he tweeted me “It doesn’t
work when I define a mouse down function”. This is what he’d written.
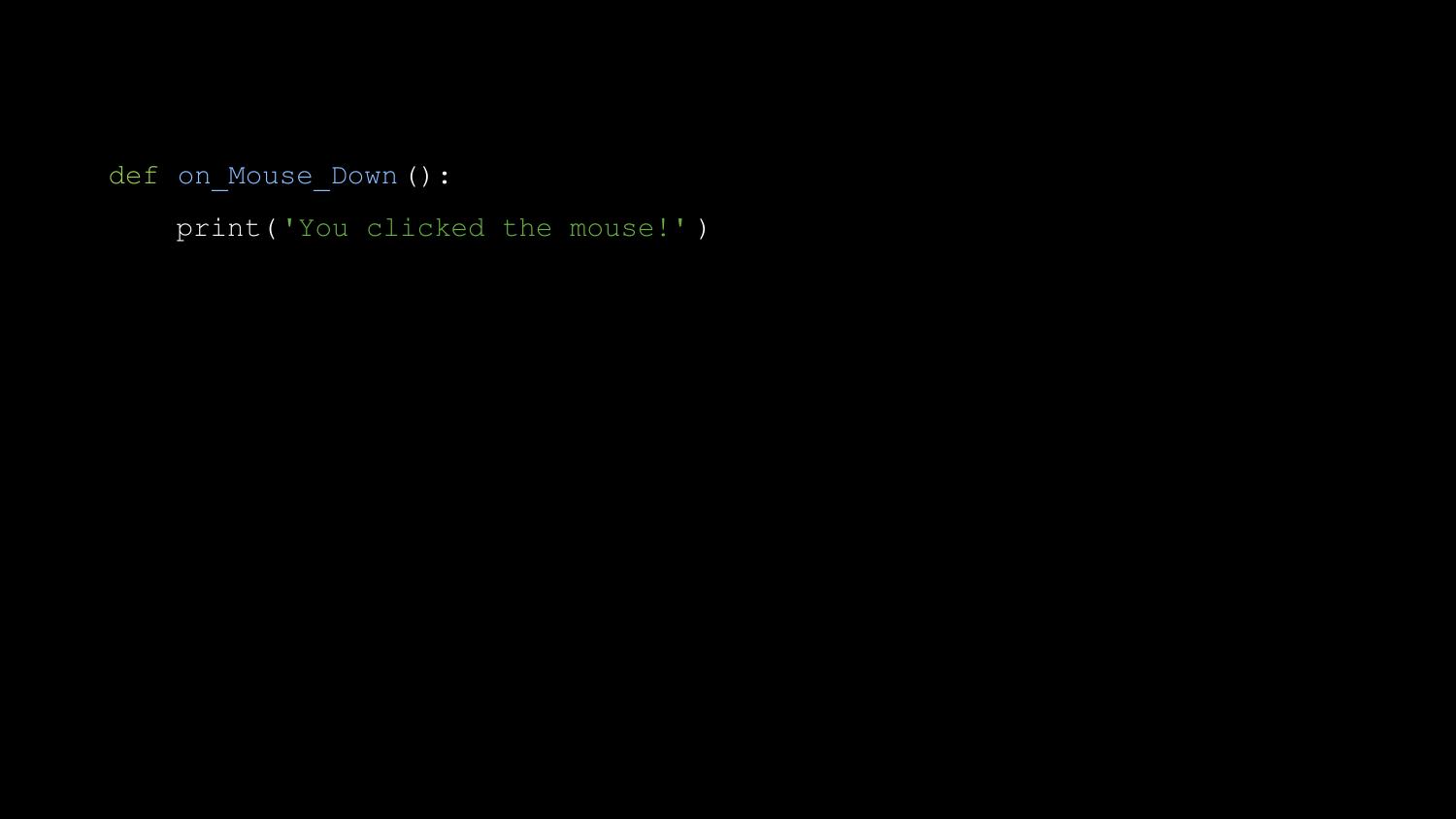
So now when you run Pygame Zero with a similar bug, it prints this:
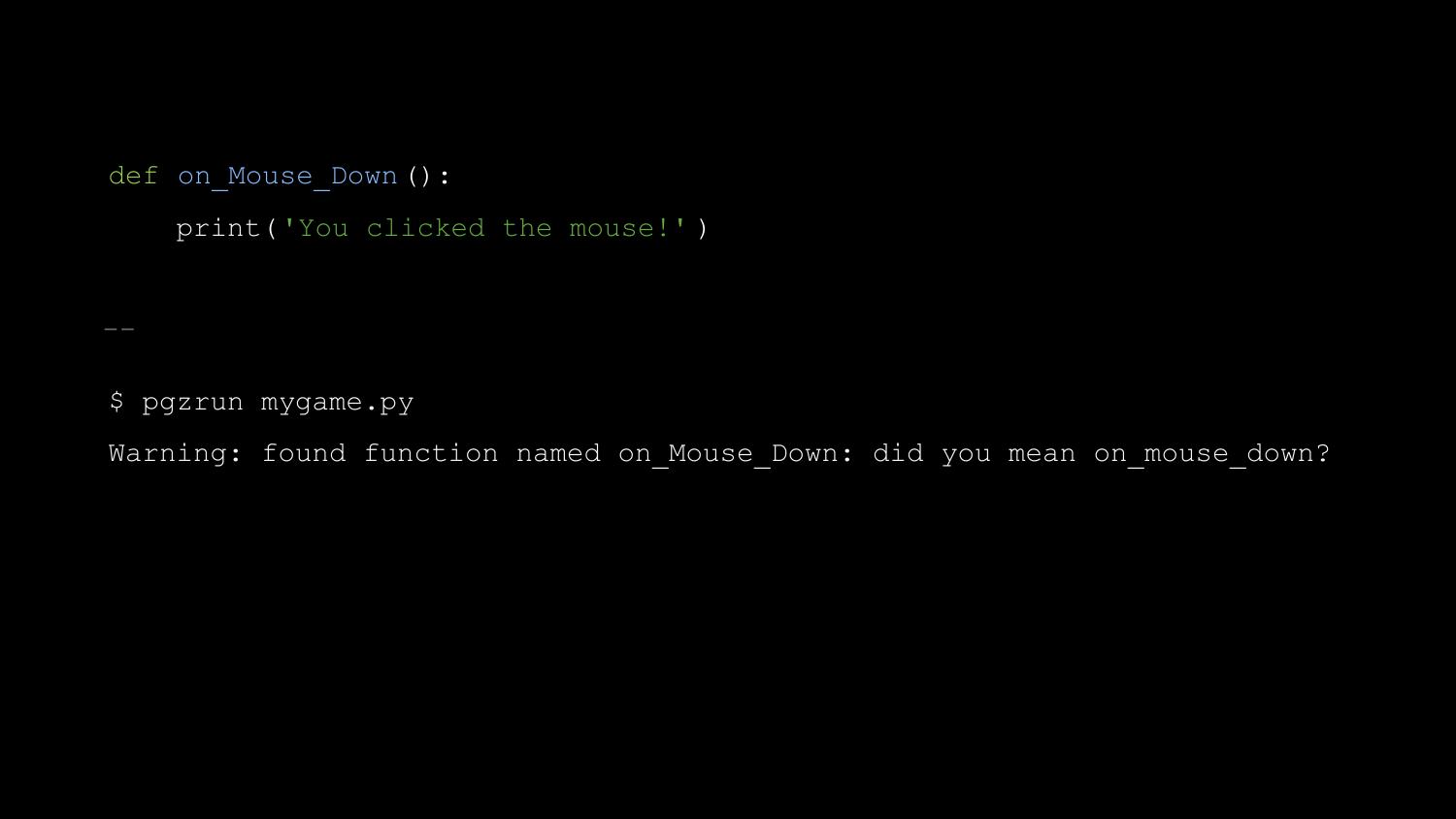
Pygame Zero was a bit of a hit - educators everywhere became very
excited about it. It ships on every Raspberry Pi, for example, and the
creator of the Raspberry Pi, Eben Upton told me “It’s pretty much
perfect.” Mark Scott, who works for the Raspberry Pi Foundation, told
me, “It has lowered the age at which we can teach text-based programming
languages by a couple of years.” (Text-based as opposed to graphical
tools like Scratch). And it spawned a bit of a spin-off movement - there
are now “Zero” libraries for GPIO, for networking, for UI, and more.
Pygame Zero is fun, and I’ve only recently realised why. Pygame Zero
lets you create games with a constant stream of reward - moments when
you get something new working - and it minimises punishment, by warning
about potential pitfalls.
I’d like to look into the future for a moment. At Europython this year,
Leblond Emmanuel announced that he has completed Python bindings for the
Godot game engine - a comprehensive, multi-platform, open-source game
engine. Roberto De Ioris has created Python bindings for Unreal Engine 4
- one of the most well known game engines.
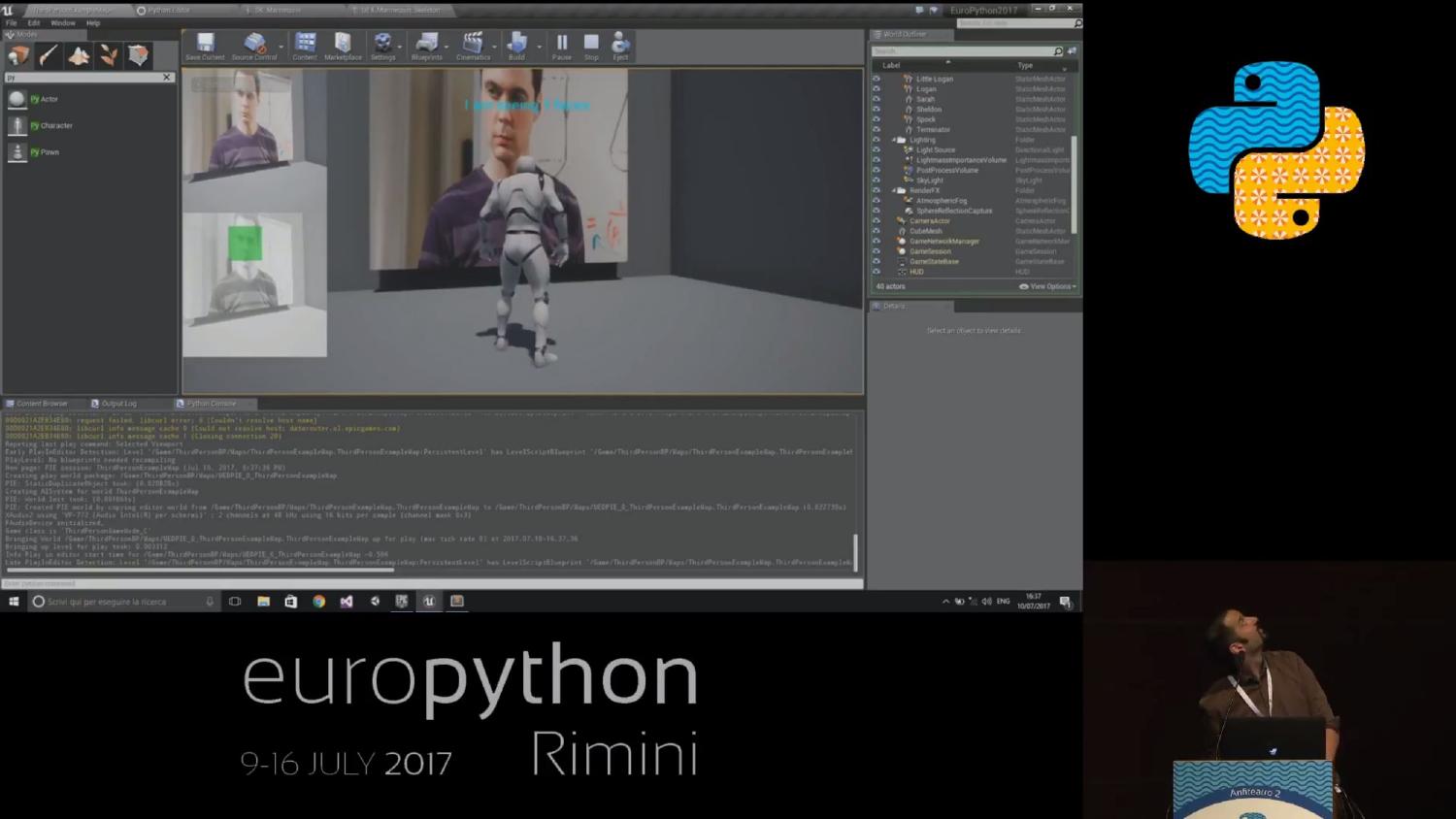
But you don’t need a huge, modern, 3D game engine. Slobodan Stevic
recently published a game called Switchcars - written with Pygame - on
Steam.
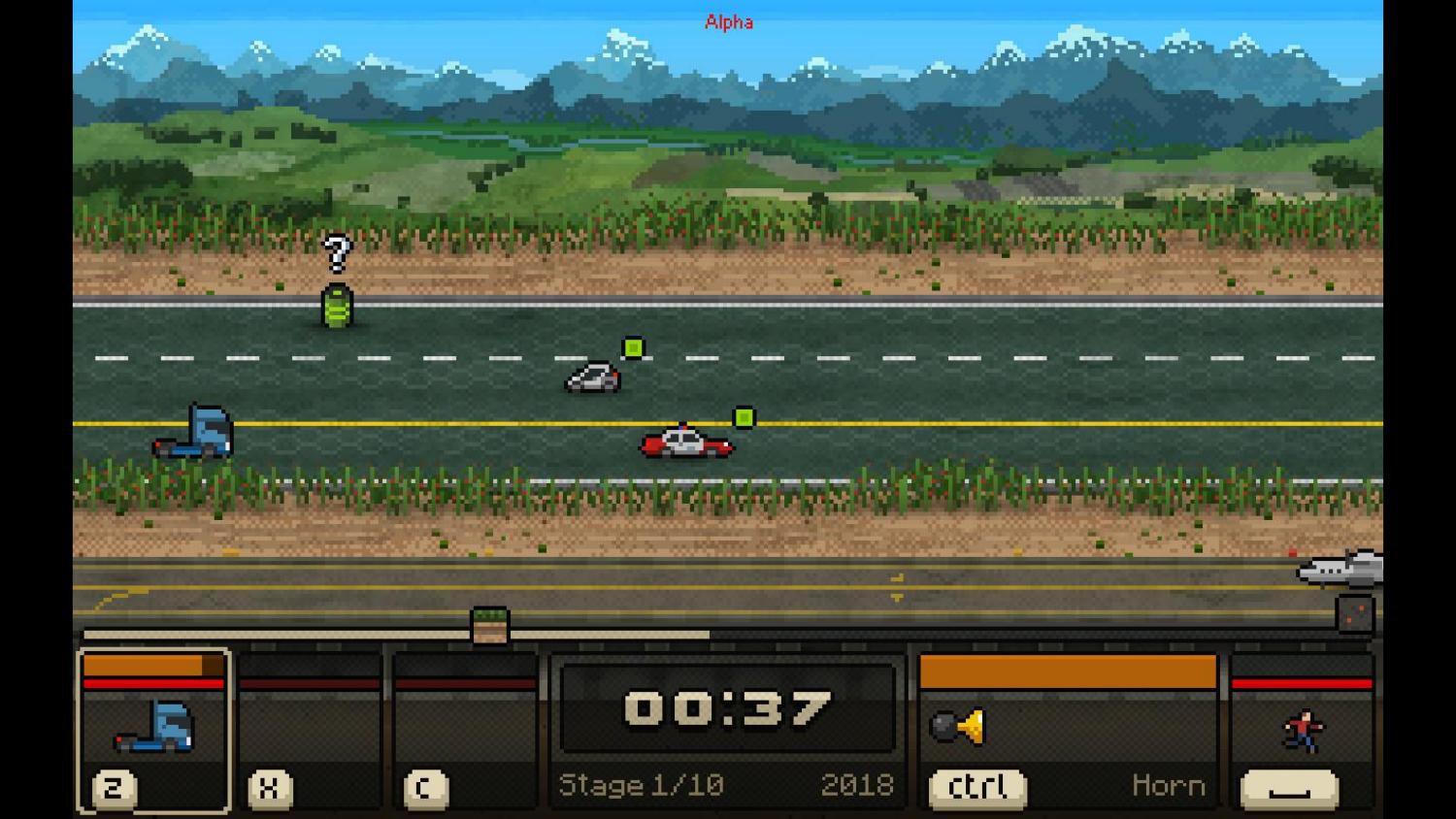
Let me try to summarise what I think this story teaches us.
Firstly, yes, you can program games in Python. You can do it today, and
you can do it commercially. Or you could just do it for fun.
Secondly, if you’re starting a new library, try to make sure Richard
Jones is there to help. But seriously - perhaps we should be a bit more
like Richard? We should try to have that infinite enthusiasm, and be
quick to help kick-start other people’s projects. If you’re starting a
new project, particularly a game or a games library, I’d like to
volunteer to help you.
Thirdly, make it fun. Whatever you’re creating, make it reward people as
much as possible, and make sure it doesn’t punish people.
Finally, please sign up for the next Pyweek in October. I’m sure you
will have fun - but you might just end up creating something amazing,
something that will make Python better for for education, or for fun, or
for all of us.
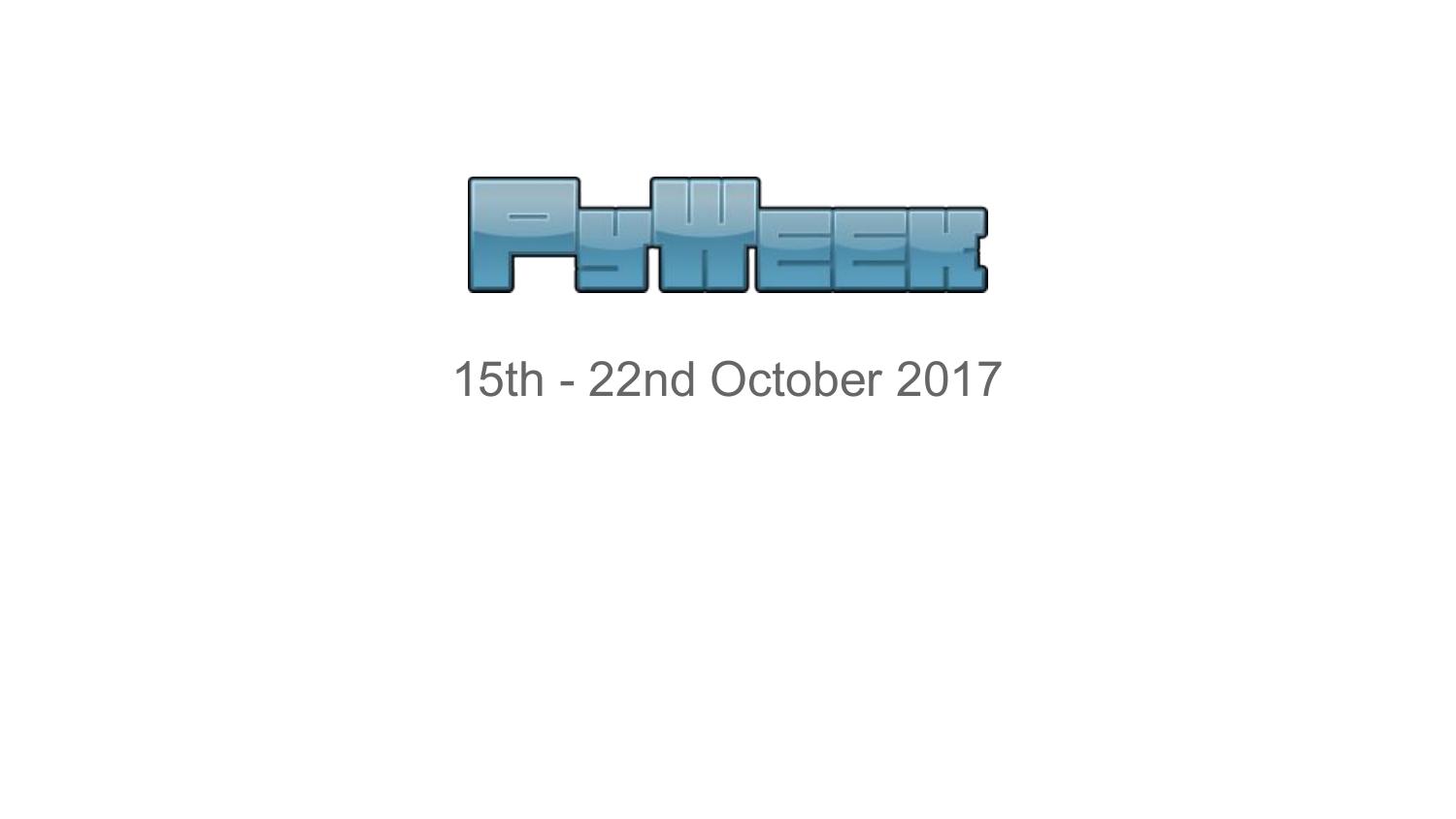
Thank you.